- Campbell Arnold
- Mar 11
- 5 min read
Updated: Mar 16
Zero-to-Hero: AI-Guided Ultrasound Enables Non-Expert Users
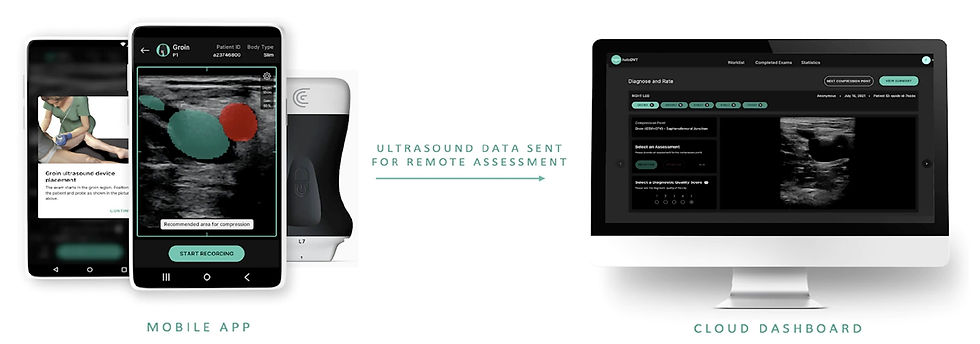
Diagnosing deep vein thrombosis typically requires an expert sonographer to perform compression ultrasound. However, there is a shortage of trained sonographers to meet a rising demand, and some healthcare sites may not have any expert sonographers on staff. A recent NPJ Digital Medicine article evaluated ThinkSono’s AI-guided ultrasound system, which is designed to enable non-expert operators to conduct DVT scans. AI provides users with real-time guidance during image acquisition, and clinicians later review the scans for diagnosis.
The study included 381 patients from 11 UK hospitals who underwent both an AI-guided scan by a non-ultrasound-trained nurse and a scan performed by an expert sonographer. Scans were collected on a portable handheld ultrasound from Clarius, and all images were reviewed by five UK radiologists and five US emergency medicine physicians with point-of-care ultrasound expertise. Overall, 80% of AI-guided scans were diagnostic quality, with clinician review demonstrating high sensitivity (90-98%) and negative predictive value (98-99%). This indicates that AI-guided acquisitions have potential as a reliable alternative to sonographer-led exams.
Using AI to enable non-experts to perform ultrasound exams also has significant economic and logistical benefits. The study found that AI-guided imaging reduced the need for expert-led scans by 29-53%, which could help alleviate workforce shortages and expand ultrasound access to locations where trained sonographers are scarce. Emergency medicine physicians performed particularly well with the system, likely due to their experience with point-of-care ultrasound. Overall, the work highlights the potential of AI to expand medical imaging access and improve DVT diagnosis in both well-resourced hospitals and underserved settings.
AI Takes the Call: Automating Emergency MRI Protocoling

A protocol consult probably isn’t most radiologists' favorite call. Unfortunately, with imaging volumes continually increasing, radiologists will have to spend even more time on this time-consuming task that interrupts their workflow. A recent Radiology: Artificial Intelligence study evaluated five models that could automate protocol selection using the referral text and benchmarked algorithm performance against two seasoned emergency radiologists.
The study included 1,953 emergency brain MRI referrals, which were classified into 12 protocols as well as with or without contrast. The authors evaluated Naïve Bayes, Support Vector Machine, XGBoost, BERT, and GPT-3.5 models. The best-performing model was GPT-3.5, which achieved an accuracy of 84% for protocol selection and 91% for contrast determination, performing on par with the emergency radiologists (80%–83% and 89%–91%, respectively). BERT also performed reasonably well, reaching 78% accuracy for protocol selection and 89% for contrast determination.
The study highlights the potential for NLP-based AI models to streamline MRI protocoling, reducing cognitive burden on radiologists and minimizing errors. Given that AI models matched the accuracy of human readers, integrating such tools into radiology workflows could improve efficiency without impacting quality.
MICCAI LISA Challenge Results: Advancing AI for Low-field MRI
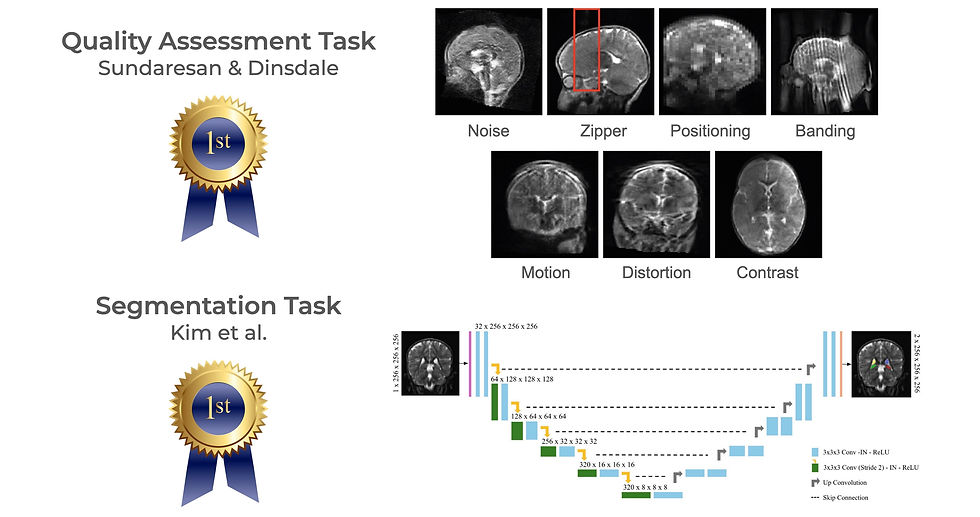
Ultra-low-field MRI is gaining traction as a cost-effective, portable imaging solution, particularly in resource-limited settings where access to traditional MRI is scarce. However, its lower image quality and lack of trained technicians and radiologists presents challenges for widespread adoption. The LISA Challenge held at MICCAI aimed to address these hurdles by developing AI-driven solutions for automated image quality assessment and hippocampal segmentation. The proceeding from the 2024 challenge were recently published in Lecture Notes in Computer Science.
The competition datasets included images from three sites in South Africa, Pakistan, and Uganda. The quality assessment task dataset contained 648 low-field images containing 7 artifact types, while the hippocampal segmentation task dataset had 99 paired high-field/low-field scans with manual segmentations. The challenge attracted 36 teams from nine countries, with top-performing approaches demonstrating the potential of AI to expand low-field MRI access. Here are some highlights and github repos for the top performing algorithms on each task:
Quality Assessment Task
DenseNet w/ Simulated Artifacts (Indian Institute of Science & Oxford University): The winning team used a DenseNet-based model to classify artifacts and achieved an 82.3% accuracy. Their training approach leveraged simulated noise and artifacts to improve robustness in detecting image quality issues.
Multi-Label MambaOut Model (Northwestern Polytechnical University): This team framed the problem as a multi-label classification task and used a Mamba model with gated convolution blocks for artifact detection. They also used a class balance loss to help with the lopsided artifact representation.
DenseNet w/ Axis Classifier Module (University of Ulsan): For quality assessment, this team implemented a DenseNet architecture with an axis classifier module to provide orientation input during quality evaluation.
Hippocampal Segmentation Task
3D nnUNet w/ multi-labels (University of Ulsan): While finishing 3rd in the QA task, this team brought home the goal in segmentation. They used a 3D nnUNet and achieved a Dice score of 0.72 with their ensemble model.
LoFiHippSeg w/ dual view learning (Monash University): Using a dual-view learning structure, this model improved segmentation by integrating complementary anatomical information. They also used high-frequency masking to boost accuracy.
nnUNet w/ external data (Shenzhen Technology University & University of Hong Kong): This team trained an nnUNet using both the high-field and low-field MRI data, as well as incorporating additional external open access data during training from the BME24 and M4RAW datasets.
Automated quality control and anatomical segmentation could make low-field MRI more practical for broad use, reducing the amount of training necessary for acquisition and interpretation and expanding imaging access to underserved regions. The success of the LISA Challenge highlights the growing role of AI in bridging gaps in medical imaging accessibility, with promising implications for future research and clinical adoption.
Resource Highlight (2 FREE conferences!)
Deep Reconstruction Workshop at JHU (Free In-Person & Virtual)
This year's FREE Deep Reconstruction Workshop will be held March 22-23rd at Johns Hopkins University, hosted by J. Webster Stayman. This event is a premier forum on deep learning-based image reconstruction across multiple medical imaging modalities, including MRI, CT, PET, and ultrasound. The workshop features a stellar lineup of invited speakers from leading institutions, and will explore state-of-the-art advancements and future directions in the field. This free event is open to both in-person and virtual attendees, but registration is required. Secure your spot before March 15 to ensure access!
NVIDIA GTC 2025 in San Jose (Free Virtual)
NVIDIA’s GTC AI Conference is happening March 17-21, bringing together the brightest minds in AI. The conference features keynotes from industry leaders, deep-dive technical sessions, and cutting-edge AI research spanning healthcare, robotics, and beyond. Whether you're interested in the latest advancements in medical AI or looking to broaden your exposure to other AI fields, GTC has something for you. Virtual attendance is completely free, but registration is required—secure your spot today!
Feedback
We’re eager to hear your thoughts as we continue to refine and improve RadAccess. Is there an article you expected to see but didn’t? Have suggestions for making the newsletter even better? Let us know! Reach out via email, LinkedIn, or X—we’d love to hear from you.
References
Speranza, Giancarlo, et al. "Value of clinical review for AI-guided deep vein thrombosis diagnosis with ultrasound imaging by non-expert operators." npj Digital Medicine 8.1 (2025): 135.
Huhtanen, Heidi J., et al. "Machine Learning and Deep Learning Models for Automated Protocoling of Emergency Brain MRI Using Text from Clinical Referrals." Radiology: Artificial Intelligence (2025): e230620.
Lepore, Natasha, and Marius George Linguraru. "Low field pediatric brain magnetic resonance image segmentation and quality assurance." 27th International Conference on Medical Image Computing and Computer Assisted Intervention (MICCAI 2024). Zenodo. 2024.
Disclaimer: There are no paid sponsors of this content. The opinions expressed are solely those of the newsletter authors, and do not necessarily reflect those of referenced works or companies.