- Campbell Arnold
- Mar 25
- 6 min read
Welcome to Radiology Access, your biweekly newsletter on the people, research, and technology transforming global imaging access. If you want to stay up-to-date with the latest in Radiology and AI, then click here to get RadAccess sent directly to your inbox!
MedHELM: Comprehensive Benchmarking for Medical LLMs
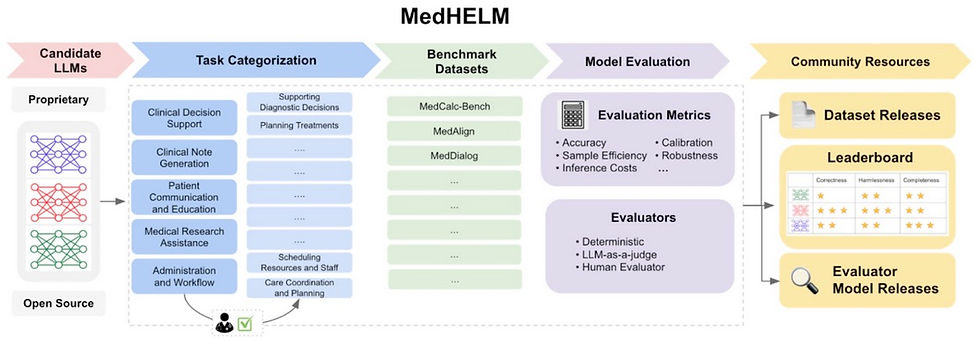
LLMs such as ChatGPT have demonstrated impressive performance on standardized medical exams like the United States Medical Licensing Examination (USMLE) and radiology board exams. However, excelling on multiple-choice tests is a far cry from practicing clinical medicine—after all, a passing STEP1 score doesn’t preclude you from killing patients! As LLM applications in healthcare expand, it’s essential that we develop more rigorous and comprehensive benchmarking methods to ensure their reliability, safety, and clinical utility. Such benchmarking tools will prove invaluable for selecting the appropriate model for a given clinical task.
Existing evaluations often focus on general language understanding, but fail to address the complexities of real-world medical practice. To bridge this gap, researchers at Stanford and Microsoft developed MedHELM, a comprehensive benchmark designed to assess LLM performance on clinically relevant tasks using real electronic health records. The MedHELM project builds upon the original Holistic Evaluation of Language Models (HELM) framework and was developed in collaboration with a large team of clinicians spanning 15 medical specialties.
MedHELM evaluates models across five key categories: Clinical Decision Support, Evidence-Based Medicine, Biomedical Knowledge, Clinical Conversations, and Medical Reasoning. These are further divided into 22 subcategories and 121 distinct tasks, ensuring a broad and clinically relevant assessment of AI performance. The benchmark draws from 31 datasets—12 private, 6 gated-access, and 13 public—capturing a wide range of medical scenarios, from diagnostic decision-making to patient communication. To ensure robustness, MedHELM employs multiple evaluation metrics, including accuracy, robustness, fairness, and bias detection, alongside real-world usability factors such as calibration and reliability in high-stakes clinical environments.
By aligning AI evaluation with real clinical needs, MedHELM represents a critical step forward in benchmarking medical AI. Crucially, this framework provides a much more nuanced understanding of an LLMs performance, including identifying individual strengths and limitations of particular models. For instance, in initial testing the authors found smaller models actually outperformed their state-of-the-art counterparts in some tasks like patient communication. These insights will be crucial in guiding AI integration into healthcare, helping clinicians and developers choose the most effective tools for different applications.
UniMed-CLIP: Improving Foundation VLMs for Medical Imaging
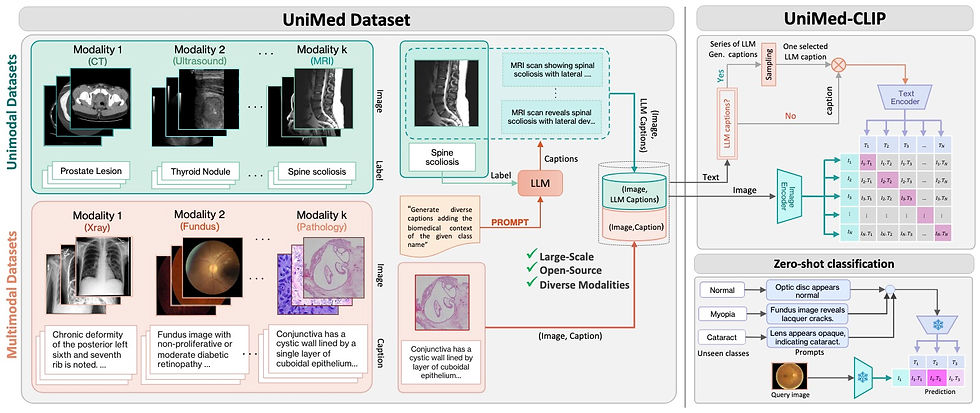
Vision-Language Models (VLMs) have recently revolutionized multi-modal machine learning by enabling models to learn joint representations of visual and textual data. These models, such as CLIP (Contrastive Language-Image Pretraining), have demonstrated remarkable performance across a wide range of tasks, including image recognition, segmentation, and retrieval. The success of VLMs in the natural image domain has spurred interest in applying similar techniques to the medical field. However, several challenges have hindered the widespread adoption of VLMs in healthcare applications.
One of the primary hurdles is the scarcity of publicly available medical image-text datasets. Unlike natural images, which can be easily scraped from the internet, medical data is heavily regulated due to privacy concerns, limiting the availability of diverse datasets. Furthermore, many existing medical VLMs are either trained on proprietary data or are specific to a single imaging modality, which restricts their generalizability across different medical domains.
Recent advancements have sought to address these limitations by developing new datasets and frameworks for pretraining medical VLMs. For instance, BiomedCLIP and MedCLIP have demonstrated that VLMs can achieve competitive performance on specialized tasks in specific modalities. However, these models still suffer from issues related to data availability, modality-specific limitations, and the scalability of pretraining processes.
A recent arXiv article introduced UniMed-CLIP, a new VLM trained using a large-scale, open-source multi-modal medical dataset, offering 5.3 million image-text pairs from six modalities: X-ray, CT, MRI, ultrasound, retinal fundus, and histopathology. To create this dataset, the authors developed a data-collection framework that uses Large Language Models (LLMs) to transform existing image-label data into image-text pairs. The resulting dataset provides a robust foundation for training generalized medical VLMs.
Using UniMed, the team trained UniMed-CLIP, a contrastive VLM that demonstrates superior performance compared to generalist VLMs and rivals modality-specific models, particularly in zero-shot evaluations across 21 imaging datasets. UniMed-CLIP demonstrated significant gains in performance, including a 12.61% improvement over BiomedCLIP despite using three times less training data. This highlights the importance of well curated datasets for training foundation VLMs for medical imaging applications and indicates VLMs can generalize well across multiple modalities while maintaining high performance. The UniMed dataset instructions, code, and model weights are all open-source and there is a demo available on Hugging Face.
Match Day 2025: Can Residency Growth Outpace the Radiologist Shortage?
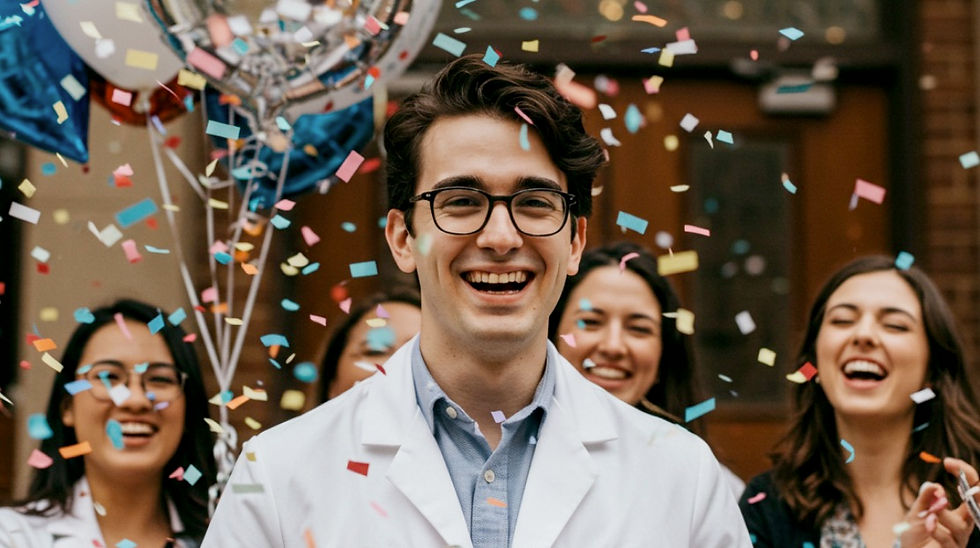
Congratulations to everyone who matched into radiology on Friday! According to Radiology Business, Match Day 2025 saw residency programs expand to an all-time high of 1,451 positions, a 5.2% increase from last year. Despite this expansion, the number of applicants to diagnostic radiology programs declined for the second consecutive year, dropping 6.4% to 1,759. This decline may be due to the specialty’s strong competitiveness in recent years, as well as concerns about AI’s impact on future job prospects. Ironically, if the perceived threat from AI discourages applicants, the resulting radiologist shortage may only increase reliance on AI to meet growing imaging demands.
Fortunately, radiology maintained a strong 98.2% fill rate, with only 26 unfilled positions (which will likely be filled after SOAP). Applicants had an 81% overall match rate, rising to 93% for U.S. MD students. While increasing residency spots is a positive step, a 5.2% annual increase will likely only keep pace with rising imaging demand rather than significantly addressing the radiologist shortage in the near term. Meanwhile, interventional radiology saw record growth, welcoming its largest-ever class of 207 residents alongside a 3% increase in applicants, reflecting continued enthusiasm for procedural radiology.
Promaxo’s Low-Field MRI System Showcases Diagnostic Potential
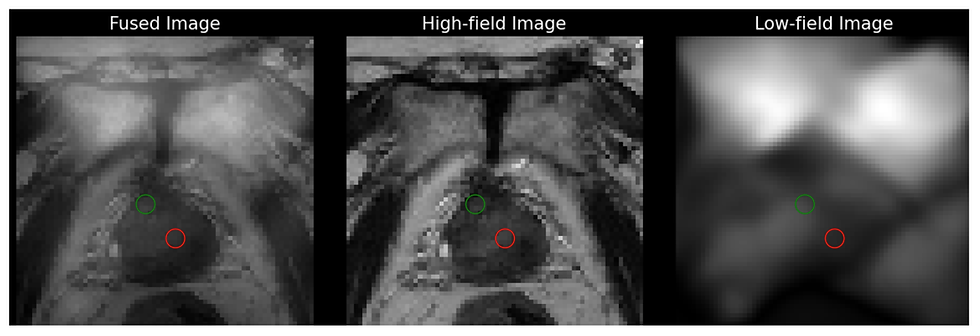
Prostate cancer is one of the most common cancers, and accurate detection is essential for guiding treatment decisions. Without a precise biopsy, there’s a risk of missing cancerous lesions or misdiagnosing benign conditions, both of which can significantly affect patient outcomes. Imaging guidance, particularly through MRI and ultrasound, plays a key role in improving accuracy. While ultrasound offers advantages in real-time guidance and accessibility, it lacks the superior soft tissue contrast and lesion visualization that MRI provides, which are crucial for more accurate cancer detection. However, high-field MRIs are costly, difficult to use for real-time guidance during procedures, and inaccessible for office-based interventions by Urologists.
To address these challenges, Promaxo has developed a single-sided low-field MRI system, designed to be used by Urologists for in-office biopsy guidance. The device lacks sufficient resolution alone, but is used alongside previously acquired high-field images via real-time registration. This system combines the anatomical targeting benefits of MRI with the convenience and accessibility of a portable office-based imaging device, offering higher accuracy than ultrasound and lower cost and better ease-of-use than high-field MRI. With over 150 units sold, Promaxo boasts a 77% cancer detection rate and a 23% increase in detecting clinically significant cancers.
In their most recent study published in Bioengineering, the authors examine whether quantitative texture features from low-field images can reliably distinguish cancerous tissue from normal tissue. The study included 21 patients with biopsy-proven prostate cancer and found significant differences in four texture metrics between cancerous and non-suspicious regions. These results align with previous research on high-field MRI, confirming that texture analysis can be successfully applied to lower-quality low-field images. By enhancing the diagnostic capabilities of low-field MRI, this work highlights its potential as a cost-effective, accessible tool for prostate cancer detection and improved biopsy planning in clinical settings.
Resource Highlight
New Radiology AI Research Journal with Limited Time Free Publication
At the start of 2025, the European Journal of Radiology officially launched a new sister journal called European Journal of Radiology Artificial Intelligence. EJR AI is an open-access journal dedicated to the meaningful application of AI in clinical radiology. The Journal is being led by Editors-in-Chief Pascal Baltzer from the Medical University of Vienna and Matthias Dietzel from University Hospitals Erlangen. Keep an eye out for publications from this new journal if you want to stay on top of radiology AI. Also, at least from my understanding of the website, there is no Article Publishing Charge (APC) for submissions made before September 29th, 2026, making it free to publish here for the next year and a half! Something to keep in mind if you want to save on publication costs.
Feedback
We’re eager to hear your thoughts as we continue to refine and improve RadAccess. Is there an article you expected to see but didn’t? Have suggestions for making the newsletter even better? Let us know! Reach out via email, LinkedIn, or X—we’d love to hear from you.
References
Kung, Tiffany H., et al. "Performance of ChatGPT on USMLE: potential for AI-assisted medical education using large language models." PLoS digital health 2.2 (2023): e0000198.
Bhayana, Rajesh, Satheesh Krishna, and Robert R. Bleakney. "Performance of ChatGPT on a radiology board-style examination: insights into current strengths and limitations." Radiology 307.5 (2023): e230582.
https://hai.stanford.edu/news/holistic-evaluation-of-large-language-models-for-medical-applications
Khattak, Muhammad Uzair, et al. "Unimed-clip: Towards a unified image-text pretraining paradigm for diverse medical imaging modalities." arXiv preprint arXiv:2412.10372 (2024).
Le, Dang Bich Thuy, et al. "Haralick Texture Analysis for Differentiating Suspicious Prostate Lesions from Normal Tissue in Low-Field MRI." Bioengineering 12.1 (2025): 47.
https://www.sciencedirect.com/journal/european-journal-of-radiology-artificial-intelligence
Disclaimer: There are no paid sponsors of this content. The opinions expressed are solely those of the newsletter authors, and do not necessarily reflect those of referenced works or companies.