- Campbell Arnold
- Sep 8, 2024
- 6 min read
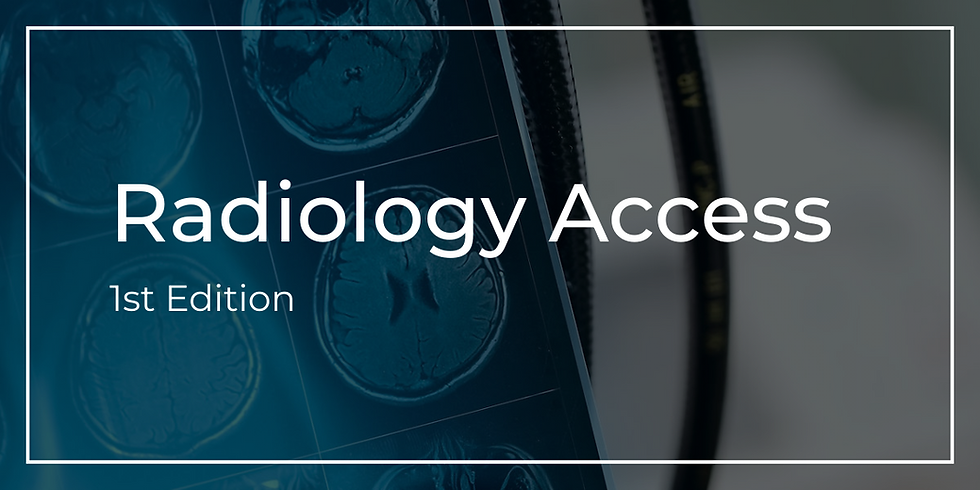
Advances in Low-Field MRI: A New(ish) Frontier in Radiology
The field of radiology continues to push on the boundaries of what’s possible with innovative techniques in low-field imaging, a burgeoning technology that could significantly impact global healthcare. In recent years, there has been a significant increase in low-field MRI research interest. The ISMRM community is leading the low-field charge, with prominent low-field researcher keynotes over the last 5 years. RSNA also continues to call for late breaking abstracts on low-field MRI and is beginning to publish more high-profile low-field works. Four recent publications shed light on groundbreaking advancements in low-field MRI, explore new methods for stroke detection, angiography, and brain morphometry, all while emphasizing cost-effective and accessible solutions.
Field-Cycling Imaging Reveals Stroke Detection as Low as 0.2 mT

The University of Aberdeen has long been a pioneer in field-cycling imaging. Unlike conventional MRI, which operates at a fixed magnetic field strength, field-cycling imaging varies the magnetic field to measure changes in the T1 relaxation time of tissues. This can reveal additional contrast mechanisms that can be useful in diagnosing certain conditions, such as ischemic stroke.
In their latest work, published in Radiology, the Aberdeen group demonstrated their prototype whole-body field-cycling imaging scanner on 9 patients with confirmed ischemic stroke. The 45 minute procedure collected single slice scans at 4 field strengths, ranging from 0.2 mT to 0.2 T. The study revealed that field-cycling imaging scans as low as 0.2 mT could identify infarct regions in stroke patients, showing hyperintense T1 regions in infarct areas. The study showed a significant correlation between decreasing field strength and infarct-to-contralateral tissue contrast ratio. The ability of field-cycling imaging to detect subtle changes in tissue properties at low magnetic fields could spur further innovations in scanner designs for stroke imaging, particularly in resource-limited settings.
First Time-of-Flight MR Angiography Below 0.1 T
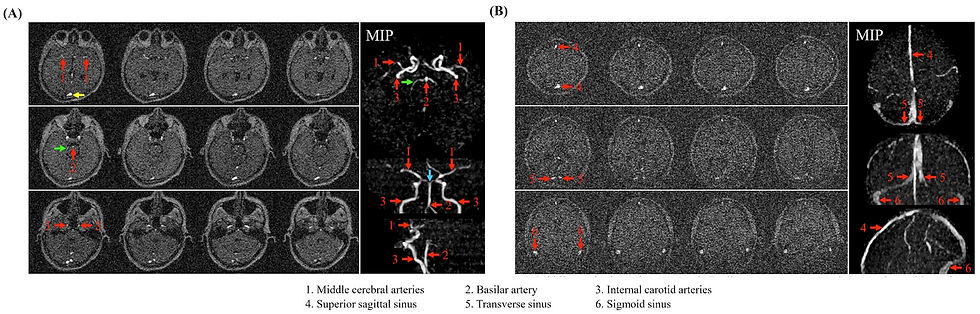
In another stride toward more accessible imaging, Ed Wu's group in Hong Kong explored the feasibility of head and neck time-of-flight (TOF) magnetic resonance angiography (MRA) using their ultra-low-field 0.05 T scanner. Their latest NMR in Biomedicine study is the first to successfully demonstrate the ability to visualize major cerebral arteries and veins using noncontrast MRA below 0.1 T.
MRA sequences are challenging at ultra-low-field given the MR low signal. To overcome this, the researchers used flow-compensated GRE sequences with a short TR to achieve strong vessel to background contrast. The sequences demonstrated potential to visualize primary brain and neck arteries and veins, though limited spatial resolution still makes small vessels difficult to see. The study is groundbreaking as it shows the potential of low-cost MR systems to perform noncontrast vascular imaging, especially in regions where high-field MRI and contrast agents are not readily available.
SAR Safe Magnetization Transfer Imaging at Ultra-Low-Field

Another study published in Magnetic Resonance in Medicine by the same Hong Kong group leveraged the low specific absorption rate in an ultra-low-field 0.055 T system for magnetization transfer imaging. Magnetization transfer imaging enhances tissue contrast, which is crucial for distinguishing between healthy and diseased tissues. However, magnetization transfer imaging requires large radiofrequency amplitudes, which can exceed specific absorption rate limits set by regulatory agencies on high-field systems.
The study showed that strong magnetization transfer effects could be achieved on a shielding-free 0.055 T scanner, even in the presence of significant B0 inhomogeneities. The researchers found that magnetization transfer effects improved lesion delineation in T2-weighted and FLAIR-like images and were robust across various tissue types, including gray matter, white matter, and muscle. The low specific absorption rate of ultra-low-field platforms allows for flexible magnetization transfer pulse design, making magnetization transfer imaging a viable and most importantly safe option for clinical imaging on low-field devices.
Reliable Ultra-Low-Field Brain Morphometry: Open Access Data Alert
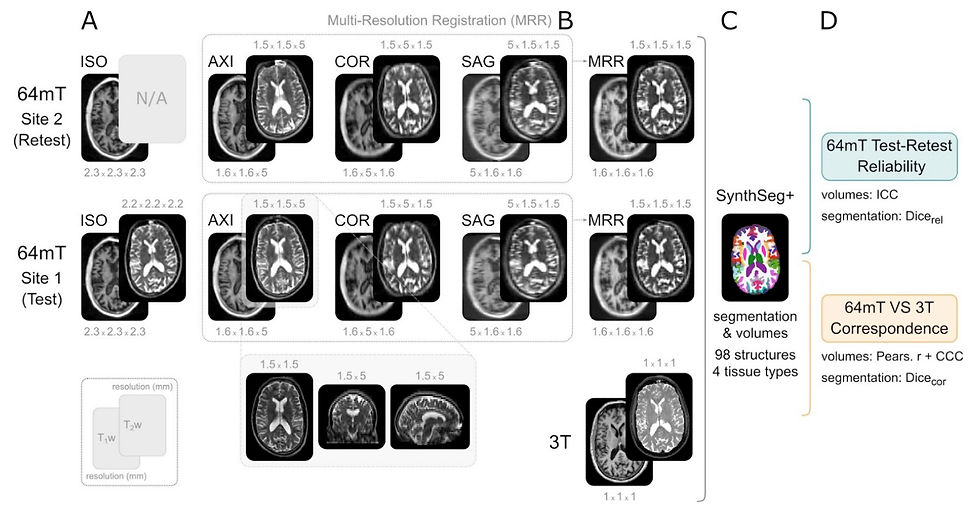
The final low-field study is a bioRxiv preprint that evaluated the potential of ultra-low-field MRI systems for brain morphometry. Brain volumes have proven to be widely applicable neuroimaging biomarkers for brain development and numerous neurological disorders. Here, researchers assessed the reliability and accuracy of morphometric measurements on Hyperfine’s 64 mT Swoop MRI, with hopes of providing a lower-cost method for assessing these pertinent biomarkers.
In the study, researchers from King’s College London scanned 23 healthy adults on two 64 mT scanners and one 3 T scanner, allowing them to probe both accuracy and reliability of low-field brain volume measurements. Results revealed high reliability between low-field measurements and strong correspondence between the ultra-low-field and high-field MRI measurements, especially for larger brain structures. The findings suggest that ultra-low-field could be a viable tool for monitoring neuroanatomical changes over time, making it particularly valuable for studying brain development and disease progression in a more accessible and cost-effective manner.
Importantly, the authors plan to release both their raw images and code upon final publication of the work. There has been substantial interest from the MRI research community in working with low-field images from this system, however to date there are not readily available open access datasets. This high quality dataset contains axial, sagittal, coronal, and isotropic T1- and T2-weighted scans from two systems, with paired isotropic 3 T scans. Researchers interested in low-field brain morphometrics and super-resolution are likely to flock to this dataset.
We will post a follow up article when the data is publicly available, so stay tuned!
Emerging Innovations in Image Interpretation
Artificial intelligence is making significant strides in image interpretation, providing tools that could potentially enhance diagnostic accuracy, reduce workloads, and improve access to the latest radiology innovations. Three recent studies explored AI applications that could reduce workloads and put the power of AI in your pocket.
Remarkably Unremarkable: AI Improves Chest Radiography Efficiency without Compromising Safety
In a fascinating Radiology study conducted across four Danish hospitals, researchers assessed the potential of an Annalise.ai algorithm (when used off-label) to streamline chest radiography workflows by automatically identifying unremarkable images.
The study involved 1,961 patients, with 1,231 unremarkable cases, and compared the AI's performance against radiologist reports. The algorithm correctly excluded pathology in 24.5% to 52.7% of unremarkable radiographs while maintaining 98-99.9% sensitivity, resulting in a 9-20% reduction in case volumes. The algorithm exhibited a similar or lower rate of critical misses compared to radiologists when set to a sensitivity of 95.4%. These findings suggest that AI could reduce the workload on radiologists without increasing the risk of diagnostic errors. However, the study emphasizes the need for prospective trials to confirm these results before widespread implementation.
Passing on PACS: Cardiac Devices Identification using Smartphones
In Radiology: Artificial Intelligence, researchers explored a method that could put the power of Radiology AI in the palm of your hand. The study focused on the development of a deep learning model for segmenting and classifying cardiac implantable electronic devices on chest radiographs.
Interestingly, while traditional DICOM images were also used, the model was predominately trained and tested using images acquired on smartphones. Their U-Net model achieved impressive results, with a mean Dice coefficient of 0.936 for segmentation and accuracies of 94% and 84% for manufacturer and model classification, respectively. This tool could assist radiologists in accurately identifying and classifying cardiac implantable electronic devices, especially in settings where smartphone connectivity is more accessible. The project’s github code is available and the data will be accessible on PhysioNet, though it’s not currently available.
A 2nd Set of A-eyes: AI Second Reader for Breast Cancer Screening
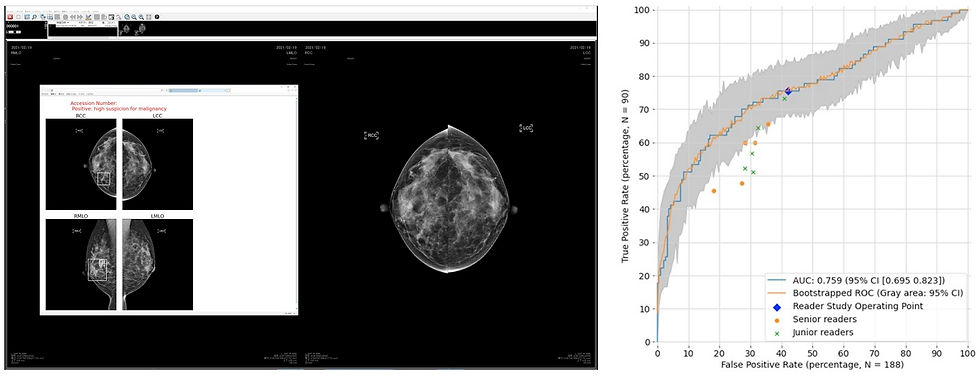
In the domain of breast cancer screening, a study explored the use of AI as a second reader in mammography. The study was published by Japanese researchers in collaboration with Google in RSNA's new open access journal, Radiology Advances. The project involved over 4,000 women and demonstrated that AI improved sensitivity by 7.6% compared to routine double reading, albeit with a slight decrease in specificity. Moreover, the algorithm reduced the need for a second reader in 71% of cases, demonstrating significant potential to decrease workloads. This suggests that AI could play a valuable role in breast cancer screening, enhancing detection rates while also streamlining the process, especially in regions facing a shortage of qualified radiologists.
Resource Highlight
Looking for more radiology news? Check out The Imaging Wire, it’s one of my favorite ways to stay updated on industry trends. This publication posts twice a week, offering an in-depth exploration of a single topic while also providing a brief overview of other notable developments. Whether you're a first year graduate student or seasoned professional, it’s a fantastic resource to keep you informed on the latest in medical imaging.
Feedback
As a new resource, we're all ears and eager to know your thoughts on how to improve our newsletter. Your feedback helps us serve you better. So, what's your take? Send us an email, or reach out to use via X.
References
Mallikourti, Vasiliki, et al. "Field-Cycling MRI for Identifying Minor Ischemic Stroke Below 0.2 T." Radiology 312.2 (2024): e232972.
Su, Shi, et al. "Ultra‐low‐field magnetic resonance angiography at 0.05 T: A preliminary study." NMR in Biomedicine (2024): e5213.
Su, Shi, et al. "Ultra‐low‐field magnetization transfer imaging at 0.055 T with low specific absorption rate." Magnetic Resonance in Medicine (2024).
Váša, František, et al. "Ultra-low-field brain MRI morphometry: test-retest reliability and correspondence to high-field MRI." bioRxiv (2024): 2024-08.
Plesner, Louis Lind, et al. "Using AI to Identify Unremarkable Chest Radiographs for Automatic Reporting." Radiology 312.2 (2024): e240272.
Busch, Felix, et al. "Open access data and deep learning for cardiac device identification on standard DICOM and smartphone-based chest radiographs." Radiology: Artificial Intelligence (2024): e230502.
Nakai, Etsuji, et al. "Artificial intelligence as a second reader for screening mammography." Radiology Advances 1.2 (2024): umae011.
Disclaimer: There are no paid sponsors of this content. The opinions expressed are solely those of the newsletter authors, and do not necessarily reflect those of referenced works or companies.