- Campbell Arnold
- Oct 8, 2024
- 6 min read
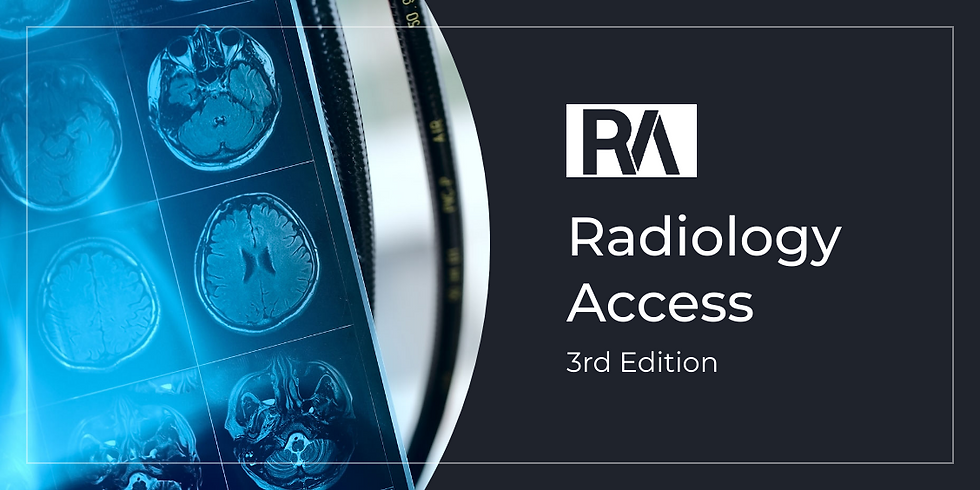
Radiology AI funding is back baby! (maybe)
It’s no secret that higher interest rates over the last two years have put a strain on venture backed startups looking to raise additional capital. In April, Signify Research reported that deal volume had hit an all-time low, with only 5 deals recorded during Q1 2024 totaling less than $50M. However, it appears that venture capitalists were eagerly anticipating the September rate cut by the US Federal Reserve.
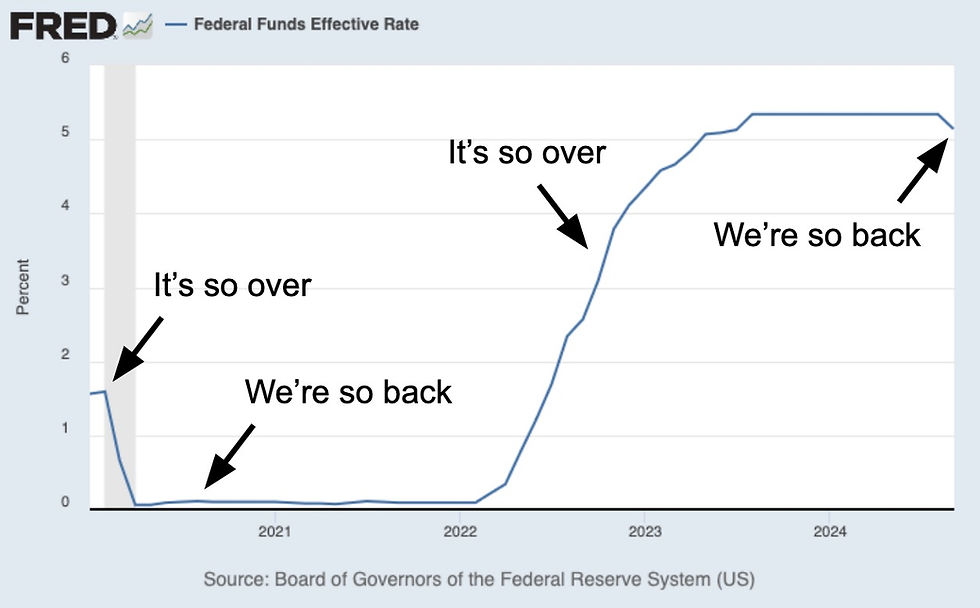
Deal volumes and sizes appeared to begin increasing around Q3, with several radiology AI companies announcing double digit raises in June, July, and August. September in particular has been a strong month, with over $170M in fund raising announced by medical imaging companies (see list below). Compare that to $627M raised in all of 2023! Deal flow for other health related AI companies also appears to be increasing. While it’s too early to declare VC funding is back, September may herald a brighter funding climate for 2025.
Investors and startups might be seeing eye to eye for a number of reasons. Investors are certainly anticipating continued rate cuts and dwindling bond returns. Additionally, after two years of declines in VC funding, cash strapped startups may be more willing to negotiate on terms. Investors may also be enticed back to the negotiation table by some recent success stories, such as the well received $410M Tempus AI IPO (NSQ: TEM).
Recent medical imaging company raises include:
Qure.ai $65M Series D (Sept 24)
SpectraWAVE $50M Series B (Sept 11)
Cerebriu $19M Series A (Sept 12)
Ferrum Health $16M Series A (Sept 3)
Segmed $10.4M Series A (Sept 3)
Perimeter Medical ~$10M non-brokered private placement (Sept 30)
DeepLook Health undisclosed Series A (Sept 18)
Aikenist undisclosed Seed Round (Oct 1)
Other recent health related AI company raises include:
Surgical robotics AI: Mendaera $73M Series B (Sept 27)
Dental AI: Pearl $58M Series B (Sept 9)
Pathology AI: PictorLabs $30M Series B (Sept 20)
Health LLM: Hippocratic AI $17M Series A extension (Sept 19)
Ophthalmology AI: Mediwhale $12M Series A2 (Oct 1)
AI for breast imaging
October is of course breast cancer awareness month, which has been accompanied by several high profile breast imaging related publications. We’ll cover two that have potential to impact patient access to high quality breast imaging and help reduce imaging workloads.
Real-time classification of benign/malignant breast tumors
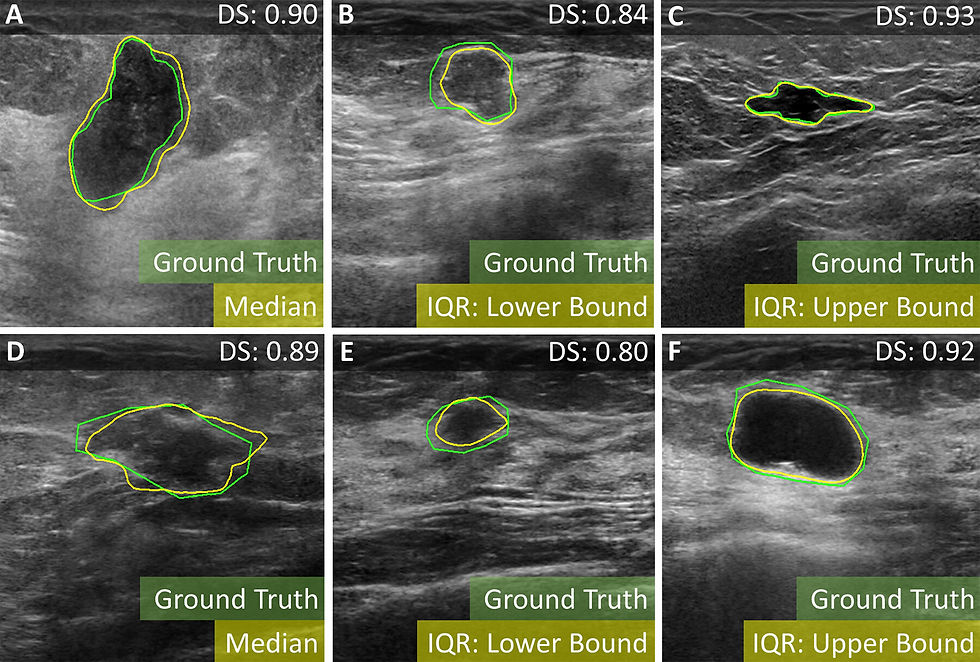
Mammography is a mainstay for breast cancer screen programs, while MRI is becoming increasingly more common. However, neither of these modalities match the broad availability of ultrasound, which could allow breast cancer screening programs to reach more people worldwide. In a recent Radiology article, researchers in Germany demonstrated a real-time method for classifying benign and malignant breast tumors on ultrasound.
Their algorithm was developed using retrospective data from almost 1200 patients, both from their institution and publicly available datasets. The authors first trained a lesion segmentation algorithm using nnU-Net. They then extracted features from the image (either whole image, segmentation only, or bounding box) using radiomics and an autoencoder. Finally, they classified the lesion (i.e. benign versus malignant) using the extracted features. The authors tested a myriad of combinations for feature extraction and classification, and found that using both radiomic and autoencoder features from tumor bounding boxes was the most effective (AUC = 0.9, sensitivity = 81%, specificity = 87%), with results on par with human radiologist performance. The researchers also demonstrated the real time capabilities of the algorithm in two cases, as shown below.
AI cuts mammography workloads in half
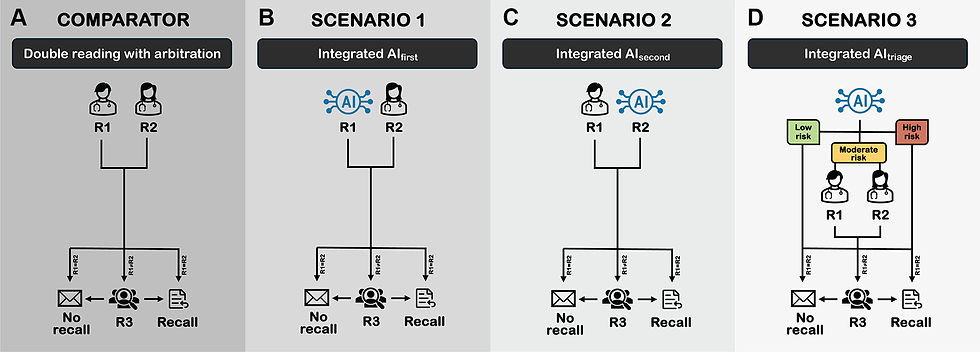
In Europe, its standard practice for mammograms to be reviewed by two separate readers. While double reading has been shown to both decrease false positive recalls and increased cancer detection rates, it comes at the cost of doubling the mammography workload. Researchers and companies have long sought to replace one or both readers with an AI system to reduce the burden.
To this aim, Danish researchers recently published a Radiology: Artificial Intelligence article evaluating different scenarios for using AI to replace the double reader system used in Europe. They retrospectively evaluated Lunit’s INSIGHT MMG system using nearly a quarter million mammograms, comparing traditional double reading to three AI implementation scenarios: AI replaces first reader (less experienced), AI replaces second reader (more experienced), or using AI to triage low, moderate and high risk cases. In short, they found using the triage approach and replacing the first, less experienced reader reduced workloads by 49-50% without impacting detection accuracy. However, replacing the second, more experienced reader did result in a sensitivity drop of -1.5%. Importantly, this study shows that AI can dramatically reduce workloads at a national scale, in this case results in over a hundred thousand less reads!
Low-field advances
Low-field MRI continues to expand its offerings, both through improved image quality and permitting imaging in notoriously difficult clinical situations. Here we’ll talk about expanding imaging access for patients in critical care settings and a recent abdominal imaging article that made the cover of Magnetic Resonance Medicine.
Low-field outperforms CT for acute brain injury in ECMO patients
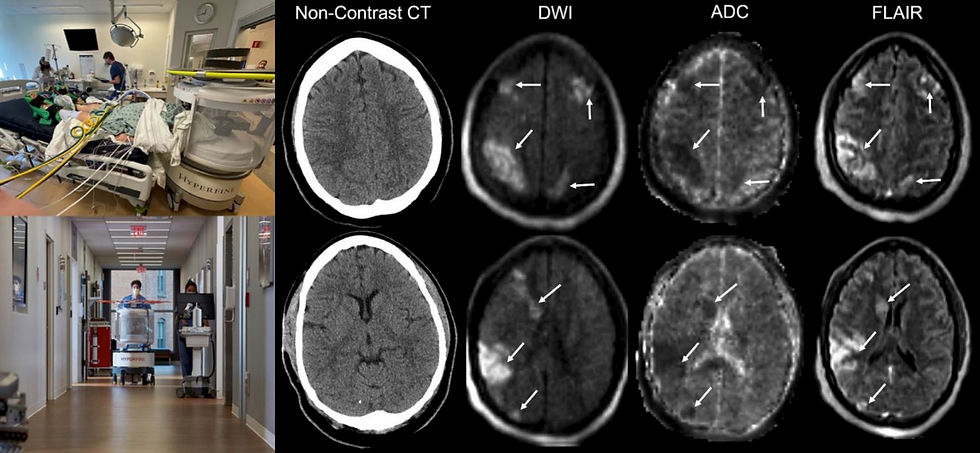
When we say someone is on life support, we often mean they’re either receiving mechanical ventilation or extracorporeal membrane oxygenation (ECMO), both of which make assessing these patients via imaging extremely difficult and risky. ECMO in particular is associated with high rates of acute brain injury, which further increases the need for imaging in these critically ill patients. Traditional high-field scanners are a non-starter with ECMO equipment, making CT the standard of care for these patients.
Options may be expanding though, in a recent Circulation article researchers at Johns Hopkins and University of Texas Houston compared the efficacy of Hyperfine’s low-field MRI and CT. The researchers scanned 50 ECMO patients at low-field, finding signs of acute brain injury in 44% of patients and experiencing adverse events during imaging of 3 patients. A subset of 18 patients received both low-field and CT scans within 24 hours, with 10 total acute brain injuries detected. Low-field detected 8 ischemic strokes and 1 intracranial hemorrhage, while CT detected 4 and 2, respectively. This study confirms that the incidence of acute brain injury is very high in ECMO patients and that low-field MRI offers better detection rates than CT.
Outstanding gains in low-field fat-suppression

Low-field also made the cover for the latest issue of Magnetic Resonance Medicine with a dramatic fat-suppression make over at 0.55T. Fat-suppression is critical in abdominal imaging, as fat can often obscure pathology. Unfortunately, at lower field strengths fat has a shorter T1 and there is less separation between fat and water signals, making fat suppression challenging.
Recently, researchers at the University of Southern California working in collaboration with Siemens Healthineers demonstrated excellent gains in low-field fat-suppression on a ramped down 0.55T Aera system. They demonstrated a 3D stack-of-spirals Dixon imaging technique that offered high-resolution and efficient fat suppression in a single 17-second breath-hold. Compared to traditional Cartesian Dixon imaging, the new approach achieved far superior spatial resolution and clearer definition of abdominal anatomy.
Resource Highlight
Are you interested in increasing radiology access? Then you’ve got to attend RAD-AID 2024. It’s an excellent one-day conference that focuses on global radiology outreach and bringing medical imaging to medically underserved populations. In the spirit of access, they keep registration costs low ($45-115). This year, RAD-AID is in Washington D.C. on November 2nd, so register soon! It’s an eye opening experience for anyone interested in radiology access, you’ll gain a whole new perspective on the complexities of expanding medical imaging to everyone, everywhere.
Feedback
As a new resource, we're all ears and eager to know your thoughts on how to improve our newsletter. Don't see an article you thought should be included? Send us an email, or reach out to use via X.
References
Venture Capital Funding for Medical Imaging AI Companies. Signify Research (April 2024)
https://www.signifyresearch.net/insights/venture-capital-funding-for-medical-imaging-ai-companies/
Magnuska, Zuzanna Anna, et al. "Combining radiomics and autoencoders to distinguish benign and malignant breast tumors on US images." Radiology 312.3 (2024): e232554.
Elhakim, Mohammad T., et al. "AI-integrated Screening to Replace Double Reading of Mammograms: A Population-wide Accuracy and Feasibility Study." Radiology: Artificial Intelligence (2024): e230529.
Tasdelen, Bilal, et al. "Improved abdominal T1 weighted imaging at 0.55T." Magnetic Resonance in Medicine (2024).
Cho, Sung-Min, et al. "Clinical Use of Bedside Portable Ultra–Low-Field Brain Magnetic Resonance Imaging in Patients on Extracorporeal Membrane Oxygenation: Results From the Multicenter SAFE MRI ECMO Study." Circulation (2024).
Disclaimer: There are no paid sponsors of this content. The opinions expressed are solely those of the newsletter authors, and do not necessarily reflect those of referenced works or companies.