- Campbell Arnold
- Nov 12, 2024
- 7 min read
Updated: Nov 12, 2024
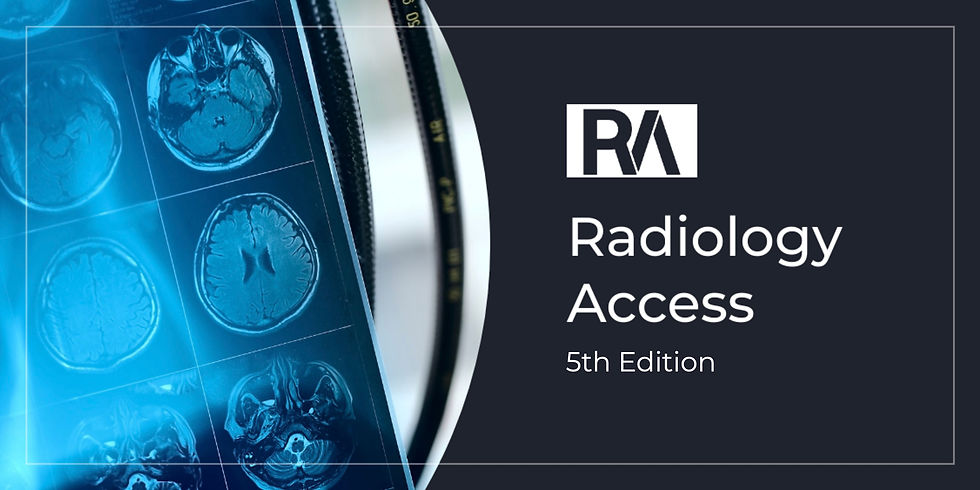
That's a wrap on RAD-AID!
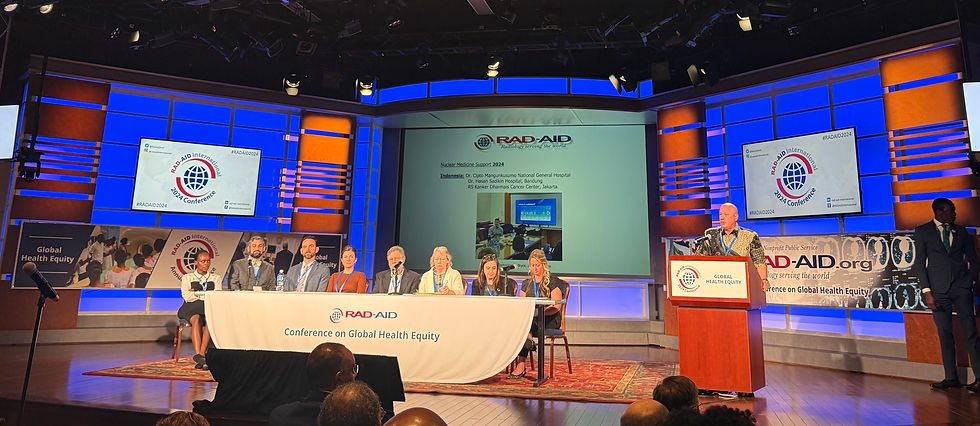
The 2024 RAD-AID Conference was held on November 2nd at George Washington University and showcased updates from AD-AID chapters and partners from around the world on their efforts to expand radiology services in resource-limited settings. Central themes of the conference included capacity building, with emphasis placed on the need for increased training access, including technologists, radiologists, and medical physicists. Updates were provided for projects from numerous RAD-AID chapters, including Nigeria, Peru, Vietnam, and Cabo Verde, as well as new fellowship programs in interventional radiology and expanded nuclear medicine facilities in west Africa. The conference emphasized the importance of integrating ultrasound, MRI, and nuclear medicine in underserved regions, with insights into the role of Mercy Ships in delivering mobile radiology services. AI and informatics was also discussed extensively, highlighting the potential for breast imaging in low- and middle-income countries, while also acknowledging the need for careful deployment and monitoring. The event concluded with a broad strategy discussion and lots of enthusiasm from participants for collaborating to expand imaging access to everyone, everywhere.
A Sensitive Issue: Low-field MRI for Multiple Sclerosis & Epilepsy
Low-field MRI is gaining traction as a more accessible imaging solution, particularly in settings where conventional high-field MRI is unavailable or overburdened. While these devices show promise for increasing accessibility, their integration into established clinical workflows remains uncertain, particularly in hospitals that already have access to high-field systems. The main limitation of low-field MRI lies in its reduced signal-to-noise ratio (SNR) and lower resolution, which can make it challenging to detect the small lesions that are crucial for diagnosing neurological conditions like epilepsy and multiple sclerosis. If patients initially assessed with low-field MRI still require follow-up imaging with high-field systems, the value proposition of these devices could be diminished. Researchers are actively exploring the diagnostic sensitivity and specificity of low-field scanners to a variety of disorders and assessing the most appropriate way to integrate these devices into clinical workflows. Two such recent studies evaluated Hyperfine's portable low-field MRI for detecting lesions in multiple sclerosis and epilepsy patients.
Low-field MRI for Epilepsy
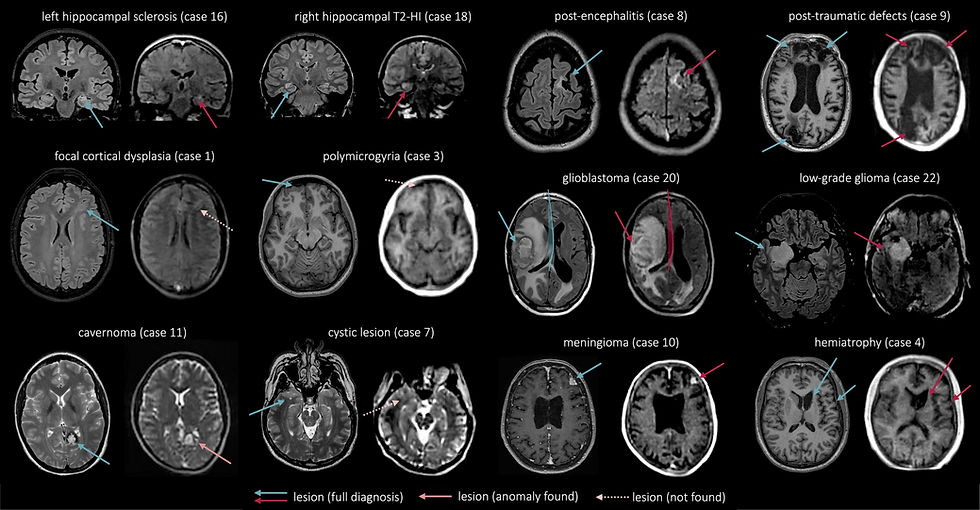
In an Epilepsia study by researchers at the University of Bonn, the authors investigate the feasibility of using a portable low-field scanner for diagnosing epilepsy brain abnormalities. Epilepsy is a complex disorder with diverse causes, ranging from large tumors to incredibly small lesions or oftentimes no visible abnormalities at all. High-field MRI (1.5T and 3T) is the gold standard for epilepsy imaging, but the high cost and infrastructure requirements of these devices limits their availability, especially in low- and middle-income countries. Even in high-resource settings, patients with epilepsy often face delays in accessing MRI scans. To address these barriers to accessibility, the authors evaluated whether a lower-cost, portable device could effectively detect structural abnormalities commonly linked to epilepsy.
Over a three-week period, the authors collected paired 3T and 64mT images from 23 patients with diagnosed epilepsy. Images from each scanner were evaluated by two different sets of neuroradiologists to ensure blinding to the patients’ clinical condition when reviewing the low-field scans. The findings revealed the low field scanner could detect 71% (17/24) of lesions with near perfect specificity and offered complete diagnostic information in 46% of cases. The authors noted the system was best suited for identifying tumors and posttraumatic lesions, while struggling more with cortical dysplasia and focal pathologies.
Low-field MRI for Multiple Sclerosis
In a similar theme, researchers in Toronto published an AJNR article evaluating the same portable MRI system for assessing key multiple sclerosis diagnostic criteria. Multiple sclerosis can be associated with very small lesions, and lesion tracking is essential for diagnosis and treatment. One of the key criteria for diagnosing multiple sclerosis is the dissemination of lesions in time and space (i.e. development of new lesions in new locations). In this study, the researchers evaluated the ability of the portable MR system to diagnose dissemination of lesions in space for patients with optic neuritis, which is a potential precursor to multiple sclerosis.
Over a year and a half, the researchers collected images from 20 patients with 3T and 64mT scanners. Two neuroradiologists, who were blinded to the clinical 3T imaging findings, assessed the low-field images for 3 lesion types (periventricular, juxtacortical, and infratentorial) and made a classification of dissemination in space using the 2017 McDonald diagnostic criteria. The portable system showed moderate accuracy for lesion detection with some variability across lesion types (periventricular = 80% [8/10], juxtacortical = 57% [4/7], and infratentorial = 38% [3/8]). When assessing patients for dissemination in space, the high-field system identified 9 cases, while the low-field system detected 5. Notably, all 5 cases identified by the low-field system matched the high-field results, indicating perfect specificity (100%), though with a lower sensitivity (56%). Importantly, the authors also tracked the impact of integrating the portable MRI into their clinical workflow and showed the device significantly reduced the time from symptom onset to MRI, from 21 days pre-deployment to 8.5 days post-deployment which could potentially enable quicker MS diagnoses and changes to management.
For both studies, while portable MRI did not match the diagnostic clarity of high-field systems, it showed potential for use as a triage tool and in settings where traditional MRI is not available. These results provide early indications for how low-field MRI can be integrated into epilepsy and multiple sclerosis patient care, particularly in settings where low-field systems are used in conjunction with conventional high-field imaging. Both studies found that low field systems have high specificity, but more limited sensitivity. The multiple sclerosis study highlights the potential of portable MRI to complement traditional systems, particularly as a triage tool for reducing care delays. Both studies also illustrate the promise for these systems as diagnostic tools where traditional imaging is not available and highlight what can be gained by further refining the technology and image quality.
Unlocking the Potential of Synthetic Image Generation
Synthetic image generation is an emerging technique in radiology that uses machine learning to create medical images, offering potential benefits such as accelerating protocols, replacing missing or corrupted sequences, and augmenting available data for model training. As researchers continue to explore this topic, we will encounter more innovative approaches and uses for synthetic data. The studies discussed below explore the potential of synthetic data to improve model generalizability and examine how different input image combinations impact synthetic image generation.
Synthetic data improves generalizability of single center models
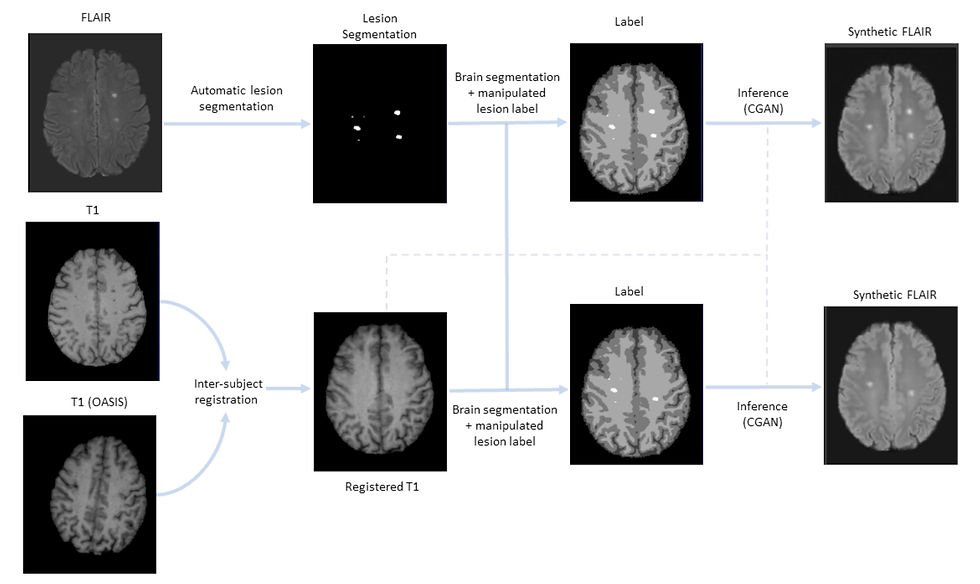
While large multicenter datasets are increasingly common, a substantial portion of models are still trained using single center data, which poses substantial challenges to model generalizability. A recent study published in Radiology: Artificial Intelligence sought to address this challenge with a synthetic data augmentation approach using generative adversarial networks (GANs) and open access data. To illustrate the potential of this approach, the authors evaluated its impact on a new lesion detection algorithm for multiple sclerosis MRI. Synthetic images are generated by introducing and manipulating lesions in brain segmentations on public images and then using those segmentations as inputs for the generative algorithm. Their code is available on Github.
The authors trained models with and without synthetic data augmentation using data from 669 multiple sclerosis patients collected at a single center. They then tested model performance using an independent dataset of 134 patients from a different institution with different scanners and protocol settings. The models that included synthetic training data showed significant improvements in performance on the external dataset (AUC: 93.3% vs 83.6%), indicating improved generalizability. This innovative approach has the potential to enhance AI model reliability not just for multiple sclerosis, but for any clinical application where pathology segmentation is available and homogeneous imaging data presents generalization challenges.
Impact of input sequences of synthetic image generation
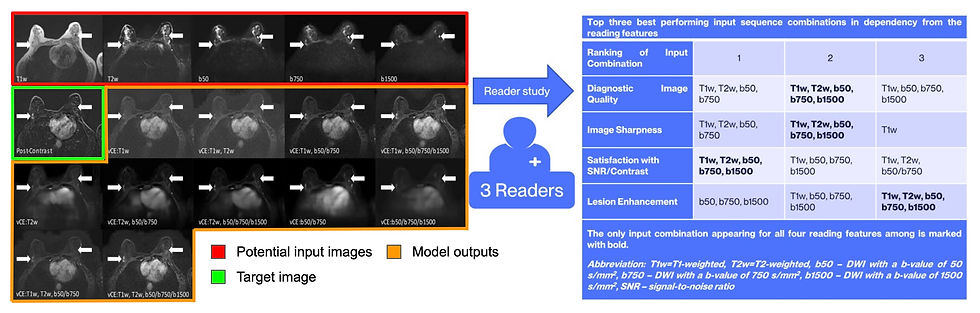
In a recent European Radiology article, researchers investigated how different combinations of non-contrast MRI sequences influence the generation of synthetic contrast-enhanced breast MRI images. Using a dataset of 1,064 multiparametric breast MRI scans, including T1-weighted, T2-weighted, and diffusion-weighted imaging (DWI) with varying b-values, the team evaluated synthetic images both quantitatively (e.g., structural similarity index [SSIM], peak signal-to-noise ratio [PSNR]) and qualitatively through assessments by three radiologists for diagnostic quality, sharpness, perceived signal-to-noise ratio, and lesion conspicuity.
The study found that unsurprisingly the highest performance was achieved by combining T1-weighted, T2-weighted, and multi-b-value DWI sequences. However, more nuanced findings also emerged. First, quantitative metrics alone were insufficient for detecting subtle differences in lesion enhancement, which underscores the importance of visual evaluations during model development. The authors noted that both T1-weighted and DWI sequences, particularly with high b-values, were essential to achieve good signal-to-noise ratio and lesion conspicuity. Despite these promising results, the researchers also highlighted a key limitation of the synthetic images, 16% of lesions had lower enhancement scores compared to traditional scans. These results emphasize the importance of qualitative review during model development and that input sequence choice is critical for successful synthetic image generation.
Resource Highlight
The RSNA Abdominal Traumatic Injury CT (RATIC) dataset is the largest publicly available collection of adult abdominal trauma CT scans, containing CT scans from 4,274 CT patients collected from 23 institutions across 14 countries and 6 continents. The dataset includes a rich set of annotations, segmentations, and image-level labels created by expert radiologists from the American Society of Emergency Radiology and Society of Abdominal Radiology. Researchers can access DICOM images, demographic data and injury details, and organ segmentations. The dataset was featured in the RSNA 2023 Abdominal Trauma Detection competition and is freely available for non-commercial use through RSNA and Kaggle platforms. Results from the 2023 Kaggle competition are also available in Radiology: Artificial Intelligence.
Feedback
As a new resource, we're all ears and eager to know your thoughts on how to improve our newsletter. Don't see an article you thought should be included? Send us an email, or reach out to us via X.
References
Bauer, Tobias, et al. Proof of concept: Portable ultra‐low‐field magnetic resonance imaging for the diagnosis of epileptogenic brain pathologies. Epilepsia (2024).
Lim, Timothy Reynold, et al. Low-Field (64 mT) Portable MRI for Rapid Point-of-Care Diagnosis of Dissemination in Space in Patients Presenting with Optic Neuritis. American Journal of Neuroradiology 45.11 (2024): 1819-1825.
Thompson, Alan J., et al. Diagnosis of multiple sclerosis: 2017 revisions of the McDonald criteria. The Lancet Neurology 17.2 (2018): 162-173.
Brugnara, Gianluca, et al. Addressing the Generalizability of AI in Radiology Using a Novel Data Augmentation Framework with Synthetic Patient Image Data: Proof-of-Concept and External Validation Classification Tasks in Multiple Sclerosis. Radiology: Artificial Intelligence (2024): e230514.
Liebert, Andrzej, et al. Impact of non-contrast-enhanced imaging input sequences on the generation of virtual contrast-enhanced breast MRI scans using neural networks. European Radiology (2024). https://doi.org/10.1007/s00330-024-11142-3
Rudie, Jeffrey D., et al. "The RSNA Abdominal Traumatic Injury CT (RATIC) Dataset." Radiology: Artificial Intelligence (2024): e240101.
Hermans, Sebastiaan, et al. "RSNA 2023 Abdominal Trauma AI Challenge Review and Outcomes Analysis." Radiology: Artificial Intelligence (2024): e240334.
Disclaimer: There are no paid sponsors of this content. The opinions expressed are solely those of the newsletter authors, and do not necessarily reflect those of referenced works or companies.