- Campbell Arnold
- Jan 20
- 4 min read
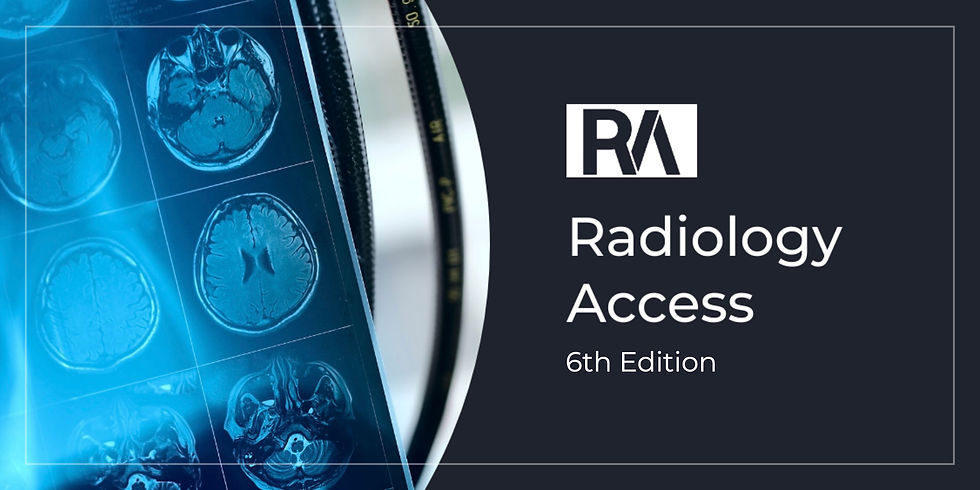
Introducing RadAccess: Impressions—your quick-read companion to the full RadAccess newsletter. Like the impressions section of a radiology report, this sub-newsletter provides a concise summary of the most important findings and their relevance. As a busy professional, sometimes you can't read the full report, but you also don't want to miss crucial details. RadAccess: Impressions delivers the main takeaways clearly and efficiently to keep you informed without the deep dive. For more details, you can always refer to the full report.
UltimateSynth: A Pan-Contrast Panacea
Ensuring robust generalization across diverse clinical settings remains a major hurdle for medical imaging AI developers. A recent bioRxiv article by Adam et al. proposed UltimateSynth, a brute force data augmentation approach that tackles generalizability by generating an extensive range of synthetic MRI sequences. UltimateSynth utilizes MR physics and tissue properties to simulate realistic MRI sequences, enabling labels from a single sequence to be seamlessly adapted to virtually any other sequence. The authors demonstrated the framework’s potential by training segmentation models on over 150,000 synthetic contrasts, achieving state-of-the-art performance and exceptional robustness across diverse datasets, scanners, and protocols. The article highlights the promise physics-informed synthetic datasets hold for enhancing AI robustness to previously unseen clinical data.
Low Field, Low Cost: Screening Neurodegenerative Disorders
Pre-clinical imaging biomarkers for neurodegenerative diseases are well established, but their use in routine screening is hindered by the high cost of imaging. In Nature Communications, researchers explored the potential of Hyperfine’s low-cost, low-field MRI scanner for detecting Alzheimer’s disease biomarkers. The authors applied advanced open-source algorithms for super-resolution image enhancement, brain region volume extraction, and white matter lesion segmentation. The study also compared isotropic and anisotropic sequences, finding that ≤3mm isotropic sequences provided greater accuracy for brain volumetrics, though scan time and motion artifacts remain important considerations. By analyzing volumetric biomarkers, the researchers successfully distinguished Alzheimer’s patients from cognitively normal individuals at the group level, demonstrating the potential of lower-cost MRI systems for Alzheimer’s disease monitoring and even screening.
Fundamental of Accelerating Low-Field Scans
While the acceleration of high-field MR sequences has been extensively studied, much less attention has been given to optimizing low-field sequences, and many high-field techniques may not translate well to the low-field regime. An arXiv publication offered a comprehensive exploration of low-field acceleration, including time-efficient data acquisition strategies, a comparison of reconstruction methods, and the "hidden noise problem." By combining k-space undersampling, scan repetition, and iterative reconstruction, the researchers optimized image quality within fixed scan durations. Among the reconstruction methods—compressed sensing, data-driven AI, and a physics-guided unrolled AI network—the physics-guided approach proved the most effective, preserving spatial fidelity and showing robustness to noise. The study also highlighted the "hidden noise problem," revealing how noisy reference images can bias quantitative evaluation metrics.
Simulated Data Powers Low-Field Denoising
While there is considerable interest in developing super-resolution algorithms for low-field scanners, there is a paucity of paired low-field/high-field datasets. This Journal of Magnetic Resonance article presents a novel approach to super-resolution algorithm development using synthetic data. The researchers created a low-field MRI denoising algorithm trained entirely on synthetic data generated using an open-source simulation tool that replicates the noise and contrast characteristics of low-field scans. The deep learning model, when applied to real-world scans, demonstrated significant improvements in contrast-to-noise ratio while preserving key structural details such as white matter hyperintensities. The main advantage of this approach is its ability to generate field-specific training datasets for various low- and mid-field systems, enabling easier adaptation of algorithms to specific scanners and enhancing generalization across different field strengths.
Accelerating Spine Sequences
One of the simplest ways to improve imaging access is by increasing the capacity of existing infrastructure through accelerated protocols. A recent Academic Radiology article evaluated the clinical effectiveness of Subtle Medical's denoising algorithm for accelerated spine MRI protocols with 40% scan time reductions. The study compared paired standard acquisitions with deep-learning-enhanced accelerated sequences for 200 patients. Radiologists found the diagnostic accuracy of enhanced accelerated images to be interchangeable with standard-of-care images, with high agreement for pathology classification. Additionally, the enhanced accelerated images achieved higher signal-to-noise and contrast-to-noise ratios across all four sequences. These findings demonstrate that deep-learning-enhanced accelerated protocols can increase scanner throughput while maintaining or even surpassing the image quality of existing standards of care.
Feedback
We’re eager to hear your thoughts as we continue to refine and improve RadAccess. Is there an article you expected to see but didn’t? Have suggestions for making the newsletter even better? Let us know! Reach out via email, LinkedIn, or X—we’d love to hear from you.
References
Adams, Rhea, et al. "UltimateSynth: MRI Physics for Pan-Contrast AI." bioRxiv (2024): 2024-12.
Sorby-Adams, Annabel J., et al. "Portable, low-field magnetic resonance imaging for evaluation of Alzheimer’s disease." Nature Communications 15.1 (2024): 1-12.
Shimron, Efrat, et al. "Accelerating Low-field MRI: Compressed Sensing and AI for fast noise-robust imaging." arXiv preprint arXiv:2411.06704 (2024).
Salehi, Aram, et al. "Denoising low-field MR images with a deep learning algorithm based on simulated data from easily accessible open-source software." Journal of Magnetic Resonance 370 (2025): 107812.
Li, Jie, et al. "Accelerated spine MRI with deep learning based image reconstruction: a prospective comparison with standard MRI." Academic Radiology (2024).
Disclaimer: There are no paid sponsors of this content. The opinions expressed are solely those of the newsletter authors, and do not necessarily reflect those of referenced works or companies.