- Campbell Arnold
- Jan 14
- 7 min read
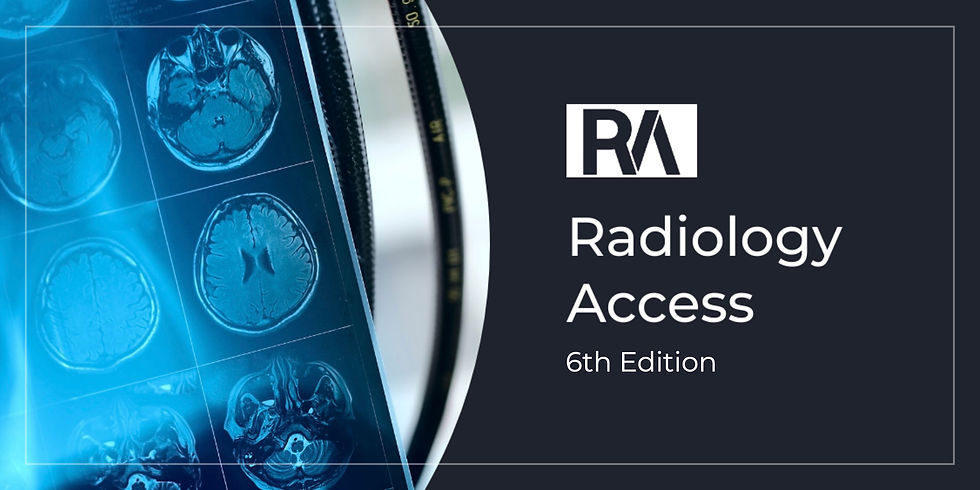
UltimateSynth: A Pan-Contrast Panacea
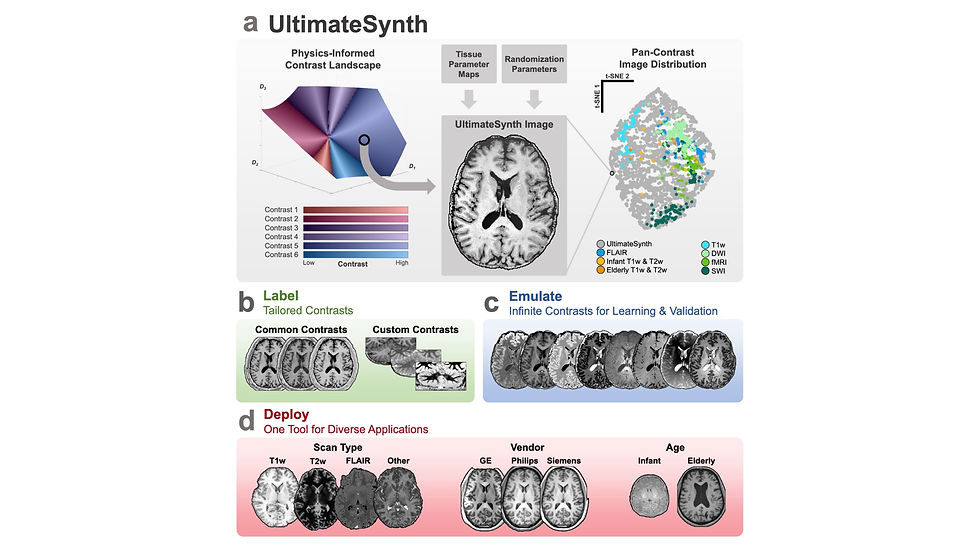
Poor generalization remains one of the biggest challenges for developers of deep learning algorithms in medical imaging. In an ideal world, we would collect infinite data—spanning all scanners, sequences, and pathologies. But data collection is already labor-intensive, and getting radiologists to label it? Forget it. To address this challenge, a recent bioRxiv publication introduced UltimateSynth, an innovative framework for generating synthetic MRI datasets with diverse contrasts.
UltimateSynth leverages fundamental MR physics and tissue properties to simulate virtually any contrast, tackling the critical bottleneck of limited sequence annotations. Unlike traditional development approaches that rely on real-world scans with fixed contrasts, UltimateSynth can produce a vast array of realistic MRI sequences. These synthetic datasets enable the creation of AI models that generalize effectively across diverse imaging scenarios.
To validate the framework, researchers trained two segmentation models on over 150,000 synthetic MRI contrasts. These models demonstrated remarkable robustness, outperforming state-of-the-art segmentation algorithms. They achieved volumetric variability of less than 2% across datasets, scanner types, patient demographics, and imaging protocols. Even when tested on external, unseen datasets, the models maintained their accuracy, underscoring the generalizability of this approach. This study showcases the transformative potential of physics-informed synthetic datasets, offering a scalable solution to advance medical imaging AI and its clinical impact.
Low Field, Low Cost: Screening Neurodegenerative Disorders
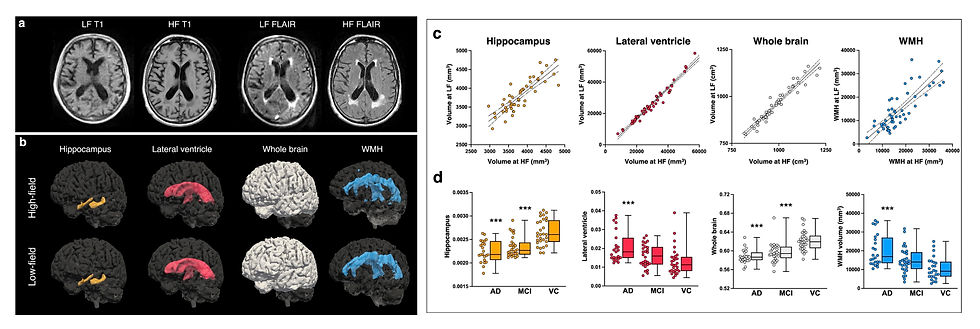
It’s well established that imaging biomarkers for Alzheimer's disease, such as brain atrophy, can precede clinical signs of Alzheimer's disease by several years. Earlier detection could allow for better patient outcomes, yet imaging biomarkers are not used for routine screening. One issue is the high cost of MRI makes image based screening prohibitively expensive and may outweigh the benefits, especially compared to other less expensive methods, like cognitive testing. A potential solution to this problem would be to lower the cost of acquiring imaging biomarkers, thus allowing for a better cost-benefit ratio.
In a recent Nature Communications study, researchers probed whether Hyperfine’s lower-cost low-field scanner was sensitive to neurodegenerative imaging biomarkers, such as white matter lesions and hippocampal volumes. To accomplish this, the authors used three impressive open source algorithms:
LF-SynthSR v2: A joint super-resolution and image synthesis algorithm that can take in any 64mT image and output a synthetic 1mm isotropic T1w MPRAGE.
SynthSeg: A robust brain segmentation algorithm that can operate on scans of any contrast, orientation, or resolution (including low-field images).
WMH-SynthSeg: Segments white matter hyperintensities on low-field T2-FLAIR sequences.
The initial version of LF-SynthSR required both T1w and T2w images as input, however this limited the tool to protocols that acquired both sequences and image quality could be impacted by poor registration. To avoid this, LF-SynthSR v2 was designed to broadly function over any single 64mT input image (i.e. any contrast, resolution, or orientation). The new version had better segmentation performance on small regions, though was slightly worse for the whole brain.
The researchers also compared conventional anisotropic sequences (1.5x1.5x5mm) to 2mm, 3mm, and 4mm isotropic sequences, finding that >=3mm isotropic sequences were more accurate for brain volumetrics. However, this doesn’t account for scan time, with 2mm isotropic sequences taking ~3x longer to collect. meaning you could acquire 3 conventional axial, sagittal, and coronal sequences in the same time. Shorter sequences are less likely to be corrupted by patient motion and can be converted into 1.5mm isotropic images using either a multi-resolution registration or machine-learning-based approach (Váša et al., 2024; Baljer et al., 2025). It would be interesting to see how these native isotropic acquisitions compared to isotropic images generated from multiple anisotropic views.
Finally, the researchers used combined low-field brain volumetrics and white matter lesion volumes to compare Alzheimer's, mild cognitive impairment, and cognitively normal patients. They demonstrated the ability to use low-field MRI biomarkers to distinguish between Alzheimer's and cognitively normal patients, at least at a group level. It will be interesting to see in future studies whether low-field biomarkers can make accurate individual level predictions about Alzheimer’s conversion.
Fundamental of Accelerating Low-Field Scans
While MRI acceleration using compressed sensing and deep learning reconstruction is well-established for high-field systems, relatively little research has explored these approaches in the low-field regime, where unique challenges like reduced signal-to-noise ratio and environmental noise can hinder performance. An international team recently published a comprehensive arXiv study, aiming to establish foundational strategies for low-field scan acceleration. The work focused on three key areas: 1) time-efficient data acquisition, 2) comparing reconstruction methods, and 3) demonstrating the “hidden noise problem.”
For time-efficient acquisition, the authors combined k-space undersampling, scan repetition, and iterative reconstruction to maximize image quality within a fixed scan duration. This approach outperformed fully sampled acquisitions and remained robust across varying SNR levels, demonstrating that strategic undersampling can achieve high-quality imaging within clinically feasible times.
The team also compared three reconstruction methods: compressed sensing, data-driven AI, and a physics-guided unrolled AI network. The physics-guided approach, which integrates MRI acquisition physics with deep learning priors, delivered the best results, preserving spatial fidelity and excelling in low SNR conditions.
Finally, the study highlighted the “hidden noise problem,” where noisy reference images can bias algorithm evaluations. By simulating datasets with controlled noise levels, the researchers showed how noisy ground truth data can inflate quantitative metrics, leading to unreliable comparisons. This insight underscores the need for rigorous benchmarking, particularly in the noisy, low SNR context of ultra-low-field MRI.
Simulated Data Powers Low-Field Denoising
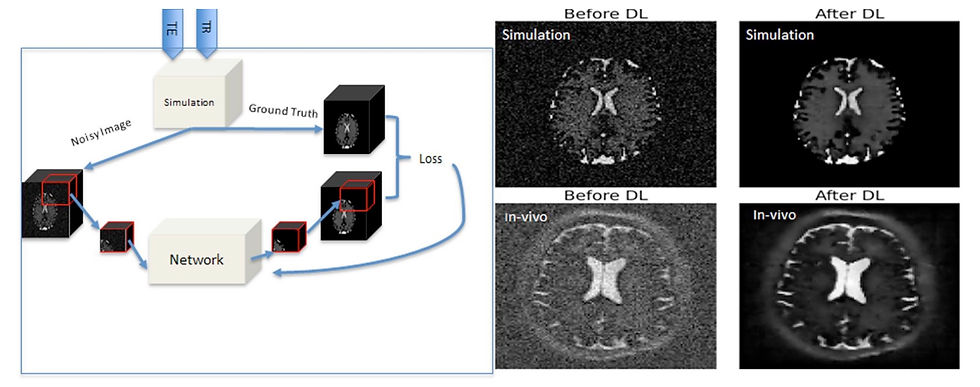
Low-field MRI has the potential to reduce imaging costs, but its lower signal-to-noise ratio continues to challenge image quality. While many researchers have applied deep learning to improve low-field image quality, most rely on paired low-field/high-field datasets, which is labor-intensive to collect and requires access to both types of scanners. For many, access to new low-field devices remains a barrier.
In a recent Journal of Magnetic Resonance article, a team from the Netherlands introduced a novel low-field MR denoising algorithm developed entirely using simulated data. Leveraging an open-source simulation tool, they generated synthetic training data that mimics the noise and contrast characteristics of real 47 mT scans, eliminating the need for paired data collection. Using the synthetic data, they trained a deep learning algorithm to perform low-field denoising and demonstrated its performance on real acquired scans. The model achieved significant improvements in contrast-to-noise ratio while preserving critical structural details, such as white matter hyperintensities.
The key innovation is the simulator’s versatility. It can generate numerous sequence types at various magnetic field strengths, allowing this methodology to adapt easily to different low-field and mid-field systems. This flexibility enables the creation of field-specific training datasets, helping to address the challenges of generalizing across different field strengths and allowing algorithms to be tailored to individual low-field scanners.
Accelerating Spine Sequences
While there is an increasing number of low-field devices, the vast majority of scanners are still high-field. This means efforts to reduce scan times for existing 1.5T and 3T scanners could significantly increase the number of patients we can service with existing infrastructure. Unfortunately, accelerated sequences typically result in noisier images that do not meet diagnostic standards. To address this, a recent prospective study published in Academic Radiology evaluated the clinical effectiveness of Subtle Medical’s denoising algorithm for accelerated spine MRI (full disclosure: my employer).
The researchers collected paired accelerated and standard acquisitions for 200 participants, with the accelerated protocols reduced scan times by approximately 40%. Quantitative analyses demonstrated that enhanced accelerated images outperform that standard-of-care, with increases in signal-to-noise ratio and contrast-to-noise ratio across all 4 sequences collected. Additionally, diagnostic accuracy was found to be interchangeable between enhanced images and standard-of-care, with high agreement among radiologists for pathology classification. The study demonstrated quantitative and qualitative evidence that the algorithm could maintain or exceed the quality of standard-of-care images while reducing scan times by 40%.
Resource Highlight
Looking for more radiology news? Check out The Imaging Wire, it’s one of my favorite ways to stay updated on industry trends. This publication posts twice a week, offering an in-depth exploration of a single topic while also providing a brief overview of other notable developments. Whether you're a first year graduate student or seasoned professional, The Imaging Wire is a fantastic resource to keep you informed on the latest in medical imaging.
Feedback
As a new resource, we're all ears and eager to know your thoughts on how to improve our newsletter. Don't see an article you thought should be included? Send us an email, or reach out to us via X.
References
Adams, Rhea, et al. "UltimateSynth: MRI Physics for Pan-Contrast AI." bioRxiv (2024): 2024-12.
Sorby-Adams, Annabel J., et al. "Portable, low-field magnetic resonance imaging for evaluation of Alzheimer’s disease." Nature Communications 15.1 (2024): 1-12.
Iglesias, Juan Eugenio, et al. "Joint super-resolution and synthesis of 1 mm isotropic MP-RAGE volumes from clinical MRI exams with scans of different orientation, resolution and contrast." Neuroimage 237 (2021): 118206.
Billot, Benjamin, et al. "SynthSeg: Segmentation of brain MRI scans of any contrast and resolution without retraining." Medical image analysis 86 (2023): 102789.
Laso, Pablo, et al. "Quantifying white matter hyperintensity and brain volumes in heterogeneous clinical and low-field portable MRI." 2024 IEEE International Symposium on Biomedical Imaging (ISBI). IEEE, 2024.
Váša, František, et al. "Ultra-low-field brain MRI morphometry: test-retest reliability and correspondence to high-field MRI." bioRxiv (2024): 2024-08.
Baljer, Levente, et al. "Ultra‐Low‐Field Paediatric MRI in Low‐and Middle‐Income Countries: Super‐Resolution Using a Multi‐Orientation U‐Net." Human Brain Mapping 46.1 (2025): e70112.
Shimron, Efrat, et al. "Accelerating Low-field MRI: Compressed Sensing and AI for fast noise-robust imaging." arXiv preprint arXiv:2411.06704 (2024).
Salehi, Aram, et al. "Denoising low-field MR images with a deep learning algorithm based on simulated data from easily accessible open-source software." Journal of Magnetic Resonance 370 (2025): 107812.
Li, Jie, et al. "Accelerated spine MRI with deep learning based image reconstruction: a prospective comparison with standard MRI." Academic Radiology (2024).
Disclaimer: There are no paid sponsors of this content. The opinions expressed are solely those of the newsletter authors, and do not necessarily reflect those of referenced works or companies.