- Campbell Arnold
- Feb 4
- 3 min read
Updated: Feb 4
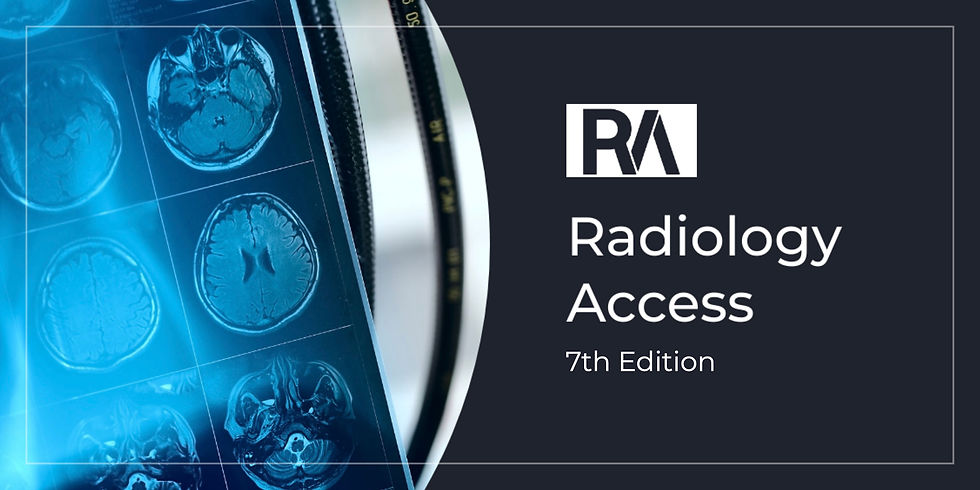
Welcome to the second edition of RadAccess: Impressions—your quick-read companion to the main RadAccess newsletter. Like a radiology report's Impressions section, here we deliver the essential information concisely, respecting your time. For more details, you can always turn to the full RadAccess newsletter.
BME-X: One model to rule them all
Image quality is often compromised by motion artifacts, noise, poor resolution, and scanner variability. While existing algorithms address some of these issues, we lack a unified approach to improving image quality. A recent Nature Biomedical Engineering study introduced BME-X, a new MR foundation model that claims to tackle all these challenges in one framework. Unlike traditional methods that map low-quality to high-quality images directly, BME-X incorporates an initial tissue classification step, providing anatomical context during enhancement. Despite using limited training data, BME-X showed robust generalization across over 13,000 images from 19 datasets, achieving state-of-the-art performance in motion correction, denoising, super-resolution, and downstream tasks. The team plans to expand its application to low-field MRI and additional sequence types, and their code is openly available.
Improving cardiac MR efficiency and accessibility
Cardiac MRI is a crucial diagnostic tool, but its complex planning can be time-consuming, error prone, and requires expertise. A recent European Radiology study evaluated Siemens Healthineers' AI-driven automated cardiac MR planning tool, demonstrating its potential to improve both accuracy and efficiency. The study found that 70.5% of AI-planned exams were error-free, compared to only 44.7% with manual planning. AI particularly excelled at reducing forgotten views and field-of-view mismanagement. Automation also improved workflow efficiency, cutting idle time by 55% and total scan time by 17% during free-breathing scans. By standardizing procedures and reducing the technical burden on technologists, AI planning could help expand access to cardiac MRI and address workforce shortages.
Low-fields, high sensitivity
Access to timely and accurate multiple sclerosis diagnosis is crucial for effective disease management, but access to high-field MRI can be limited, particularly in certain geographic locations and healthcare settings. NIH and University of Pennsylvania researchers published a Journal of Neuroimaging article evaluating Hyperfine’s portable ultra-low-field MRI as a potential alternative for MS diagnosis in settings where high-field MRI is inaccessible. In 55 multiple sclerosis patients, radiologists found that low-field MRI achieved 100% sensitivity and positive predictive value for detecting at least one white matter lesion, with strong accuracy in periventricular regions. While false positives were more common in juxtacortical areas due to lower signal-to-noise ratio, radiologist training and additional imaging views significantly reduced errors. These findings suggest that with proper training, low-field MRI could serve as a valuable triage tool, helping address scanner backlogs and expanding access to MS diagnosis.
Lowering language barriers
Accurate translation of medical information, including radiology reports, is increasingly critical in our interconnected world. Physicians and patients are increasingly turning to large language models (LLMs) for translation, yet their performance in this specialized domain remains largely unexplored. A recent Radiology study addressed this gap by evaluating medical translation performance for ten LLMs, including variants of GPT (OpenAI), Llama (Meta), Mixtral (Mistral AI), Qwen (Alibaba), and Yi (01 AI). The researchers translated 100 English radiology reports into nine other languages (and back again), assessing translation accuracy, clarity, and consistency. GPT-4 performed best overall, particularly for German, Greek, Thai, and Turkish. While most models handled readability well, medical terminology accuracy remained a challenge, especially in low-resource languages. Larger models and those trained on the target language performed better, but further refinement is needed to ensure precise medical translations for clinical use.
Feedback
We’re eager to hear your thoughts as we continue to refine and improve RadAccess. Is there an article you expected to see but didn’t? Have suggestions for making the newsletter even better? Let us know! Reach out via email, LinkedIn, or X—we’d love to hear from you.
References
Sun, Yue, et al. "A foundation model for enhancing magnetic resonance images and downstream segmentation, registration and diagnostic tasks." Nature Biomedical Engineering (2024): 1-18.
https://theimagingwire.com/2025/01/22/software-enabled-single-click-cardiac-mri/
Glessgen, Carl, et al. "Automated vs manual cardiac MRI planning: a single-center prospective evaluation of reliability and scan times." European Radiology (2025): 1-10.
Okar, Serhat V., et al. "High‐Field‐Blinded Assessment of Portable Ultra‐Low‐Field Brain MRI for Multiple Sclerosis." Journal of Neuroimaging 35.1 (2025): e70005.
Meddeb, Aymen, et al. "Large Language Model Ability to Translate CT and MRI Free-Text Radiology Reports Into Multiple Languages." Radiology 313.3 (2024): e241736.
Disclaimer: There are no paid sponsors of this content. The opinions expressed are solely those of the newsletter authors, and do not necessarily reflect those of referenced works or companies.