- Campbell Arnold
- Jan 28
- 5 min read
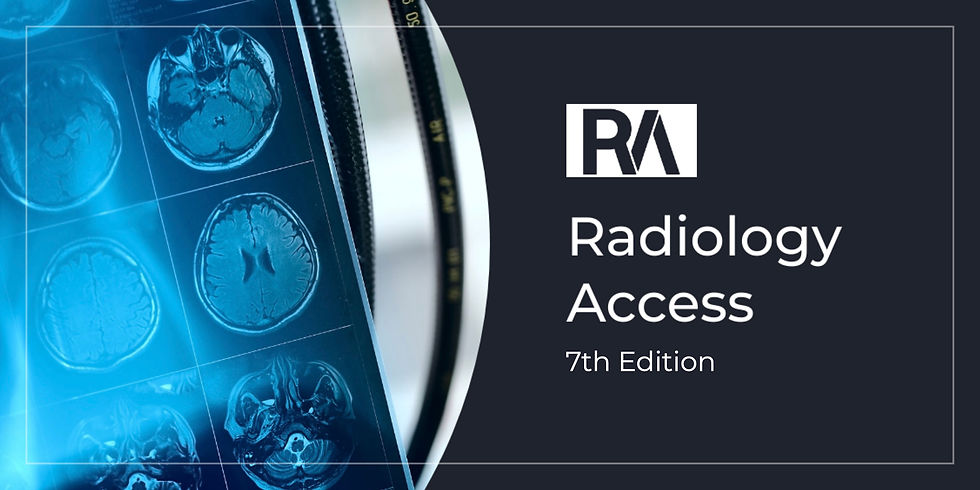
BME-X: One model to rule them all
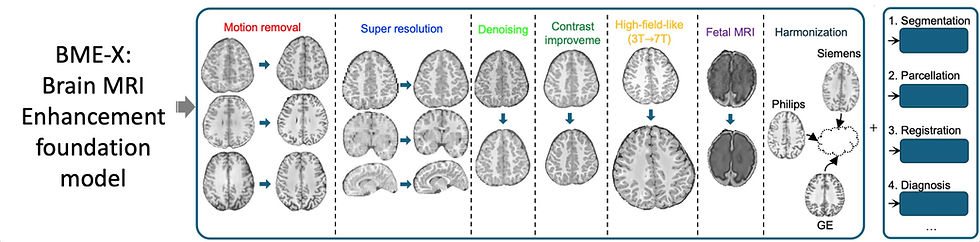
MRI image quality can suffer for a variety of reasons, including motion artifacts, noise, low resolution, and scanner variability. Most algorithms address only one or two of these issues, but in a recent Nature Biomedical Engineering article researchers at the University of North Carolina introduced BME-X, a foundation model that claims to tackle them all—offering motion correction, sharpness enhancement, denoising, contrast adjustment, and even data harmonization!
Most image enhancement algorithms learn a mapping between low-quality and high-quality image pairs directly. BME-X introduces an intermediate tissue classification module, concatenates the segmentation result to the low-quality image, and then trains a tissue-aware enhancement network. This innovative yet straightforward framework delivered impressive results across 13,000 images from 19 public datasets. Astoundingly, the researchers trained their seven age-specific models using just over 500 fetal and pediatric subjects (ages 0–6), with only 94 subjects used to develop the model they applied to validation patients ranging from 24 months to 100 years old! To make the most of their limited data, the authors used a patch-based training approach and relied on heavy data augmentation, including simulating motion corruption, noise, and blurring.
BME-X consistently outperformed other state-of-the-art methods, demonstrating robust performance across various imaging challenges, including removing motion artifacts, Gaussian and Rician denoising, and super-resolution. The authors showcased their framework's potential to generate 7T images from 3T scans and demonstrated their models preservation of pathology in multiple sclerosis and gliomas patients. As an added bonus, the authors also found BME-X improved scan harmonization across vendors. Finally, the authors demonstrated the positive impact of their foundation model on downstream tasks like tissue segmentation, registration, and diagnosis.
BME-X is an ambitious step toward a unified solution for many MRI challenges, and I’ll be eagerly awaiting future developments. The authors noted that they plan to extend the framework to additional sequence types using simulated approaches, similar to our lead article from our last edition. They also intend to apply their methods to enhance low-field Hyperfine scans. Finally, we can certainly expect to see the segmentation task expanded to include additional tissue types and pathology. Fortunately, both the code and the data for generating their foundation model is openly available.
Improving cardiac MR efficiency and accessibility
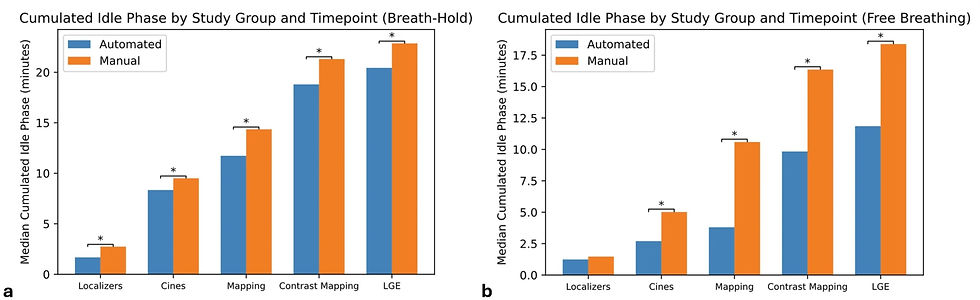
While many AI algorithms have been developed to enhance or diagnose medical images, relatively few have been designed to improve the acquisition process. Highlighted in the Imaging Wire, a recent European Radiology study evaluated the impact of AI automated cardiac MRI planning software from Siemens Healthineers relative to traditional manual planning. The researchers conducted a prospective, single-center trial with 82 patients who were randomized into two groups: AI-based automated planning or manual planning by trained technologists.
The findings revealed that automated planning significantly reduced procedural errors, with 70.5% of AI-planned exams being error-free compared to only 44.7% of manual planned studies. Automated scans particularly excelled in reducing forgotten views and field-of-view mismanagement. The study also highlighted efficiency gains from automation, particularly during free-breathing scans, where idle time was reduced by 55% and total scan time by 17%.
These types of algorithms can help standardize procedures, improving data consistency and reducing variability across technologists. Additionally, cardiac MR is a complex procedure and lowering the technical burden for planning could enable more sites to offer these scans. In a related development, UCSF and GE HealthCare recently announced a joint initiative to create a fully automated MRI service line, including high-quality remote scanning and quantitative analysis. Hopefully as more scanner manufacturers develop similar technology, we can expand access to high quality care and combat radiology workforce shortages.
Low-fields, high sensitivity
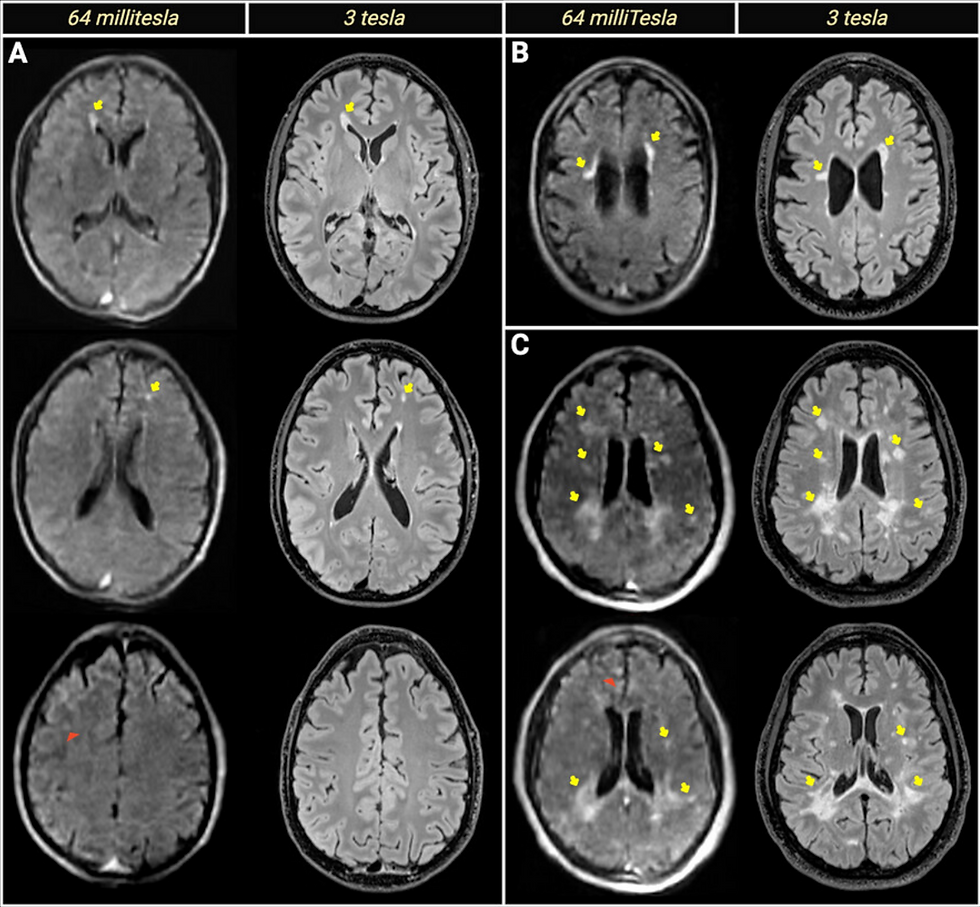
Multiple sclerosis (MS) diagnosis and treatment relies heavily on MRI to detect white matter lesions, but the high cost and limited accessibility of conventional high-field systems can delay diagnosis and start dates for disease modifying therapies. A recent Journal of Neuroimaging article evaluated the potential of Hyperfine’s portable ultra-low-field system as a diagnostic option in clinical scenarios where high-field systems are not accessible.
In the study, 55 MS patients underwent both low-field and high-field scans, with radiologists performing a blinded evaluation of white matter lesion detection. The researchers found that low-field achieved 100% sensitivity and positive predictive value for detecting at least one lesion at the case level, with particularly high accuracy in periventricular regions. However, false positives were more common in juxtacortical regions, likely due to reduced signal-to-noise ratio impacting tissue differentiation. The researchers also found that radiologist training significantly reduced false positives and improved inter-reader alignment. Additionally, adding an extra T2-FLAIR imaging plane further reduced false positives, albeit with a slight decrease in true-positive detections.
These findings show that portable low-field systems can reliably identify periventricular white matter lesions, but also highlight that training and conservative interpretation are needed to minimize false positives. With proper training, low-field systems may serve as valuable triage tools in settings with scanner backlogs or limited MRI access.
Lowering language barriers
In an increasingly global society, both physicians and patients are faced with cultural communication barriers. Luckily, large language models offer a potential solution to overcome language barriers in healthcare. A recent Radiology article evaluated the ability of various LLMs to accurately translate complex medical reports across multiple languages. The study covered ten LLMs, including variants of GPT (OpenAI), Llama (Meta), Mixtral (Mistral AI), Qwen (Alibaba), and Yi (01 AI). The authors evaluated models for their accuracy and quality in translating 100 radiology reports from English to Chinese, French, German, Greek, Italian, Russian, Swedish, Thai, and Turkish. Additionally, reports were translated back to English for evaluation.
The researchers found that GPT-4 performed the best overall, especially in translating reports from English to German, Greek, Thai, and Turkish. While all models scored fairly well on clarity, readability, and consistency, they shared a common struggle with grammar/syntax and accuracy of medical terminology. As one would expect, larger models and those trained with data from the test language tended to perform better. Overall, LLMs show promise for translating radiology reports, but further development is needed to improve the accuracy of medical terminology, particularly for low-resource languages.
Resource Highlight
Interested in pursuing a PhD in low-field MRI? King’s College London, a leading institution in low-field MRI research, is accepting applications to study low-field MRI in dementia patients. You'll be mentored by Ashwin Venkataraman, František Váša, and Steve Williams—the authors behind the feature article in the very first edition of RadAccess! This is an excellent opportunity to explore low-field MRI in depth, but if you want to apply don’t wait too long—applications close February 14th!
Feedback
We’re eager to hear your thoughts as we continue to refine and improve RadAccess. Is there an article you expected to see but didn’t? Have suggestions for making the newsletter even better? Let us know! Reach out via email, LinkedIn, or X—we’d love to hear from you.
References
Sun, Yue, et al. "A foundation model for enhancing magnetic resonance images and downstream segmentation, registration and diagnostic tasks." Nature Biomedical Engineering (2024): 1-18.
https://theimagingwire.com/2025/01/22/software-enabled-single-click-cardiac-mri/
Glessgen, Carl, et al. "Automated vs manual cardiac MRI planning: a single-center prospective evaluation of reliability and scan times." European Radiology (2025): 1-10.
Okar, Serhat V., et al. "High‐Field‐Blinded Assessment of Portable Ultra‐Low‐Field Brain MRI for Multiple Sclerosis." Journal of Neuroimaging 35.1 (2025): e70005.
Meddeb, Aymen, et al. "Large Language Model Ability to Translate CT and MRI Free-Text Radiology Reports Into Multiple Languages." Radiology 313.3 (2024): e241736.
Disclaimer: There are no paid sponsors of this content. The opinions expressed are solely those of the newsletter authors, and do not necessarily reflect those of referenced works or companies.