- Campbell Arnold
- Feb 11
- 5 min read
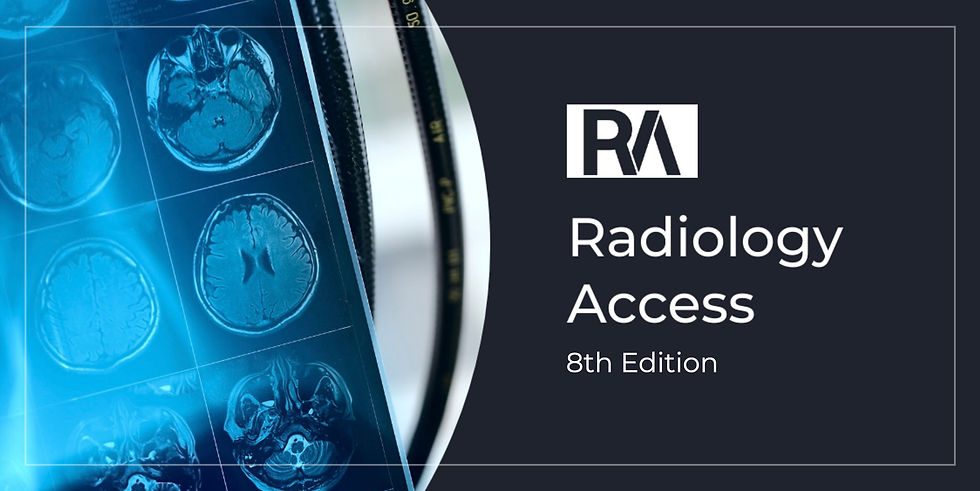
Microsoft’s Open-Access Foundation Model: BiomedCLIP
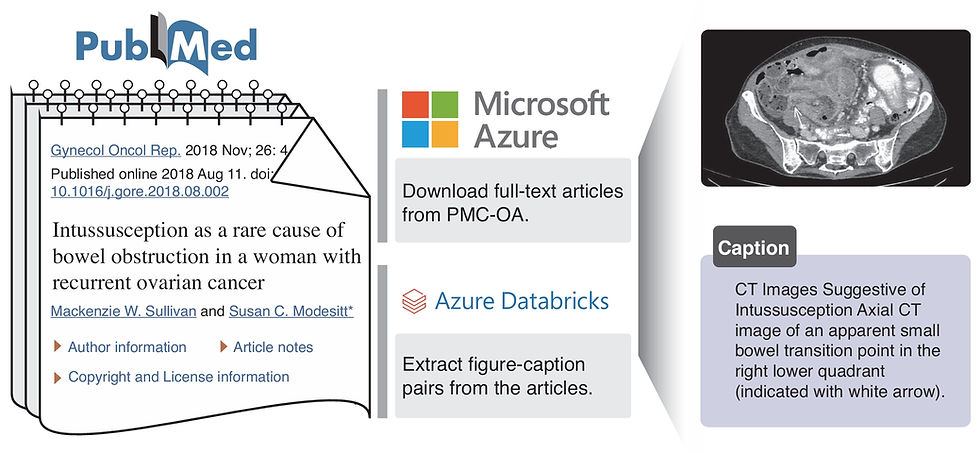
In recent years, Microsoft has put out a number of foundation models in the biomedical space, including large language models such as PubMedBERT and BioGPT as well as the image-based model suite we covered in RadAccess: 4th Edition, comprised of MedImageInsight, MedImageParse, and CXRReportGen. Microsoft continues to expand its footprint in biomedical AI with the release of BiomedCLIP, a vision-language model introduced in a recent New England Journal of Medicine: Artificial Intelligence article.
BiomedCLIP builds upon OpenAI’s CLIP (contrastive language–image pretraining) framework but is trained specifically for biomedical applications. While CLIP was developed using 400 million general-domain image-text pairs, BiomedCLIP leverages a biomedical-specific dataset of over 15 million image-text pairs extracted from 3 million open-access PubMed articles—by far the largest dataset of its kind. The model spans 30+ imaging categories, enabling it to outperform existing vision-language models across image retrieval, classification, and medical question-answering tasks. Notably, despite being a general biomedical model, BiomedCLIP outperformed radiology-specific models in challenges such as RSNA’s pneumonia detection task, demonstrating the power of large-scale, diverse biomedical pretraining.
BiomedCLIP has the potential to enhance the development cycle of radiology AI applications by reducing the need for extensive labeled datasets and manual curation. The model's impressive ability to perform zero-shot and few-shot learning enables it to generalize across different applications and imaging modalities, reducing the need for large amounts of annotated data to achieve state-of-the-art performance. By making the dataset and model openly accessible, this work paves the way for future advancements in multimodal biomedical AI, with implications both in radiology and healthcare more broadly.
MRAnnotator: Segmenting the Big Picture
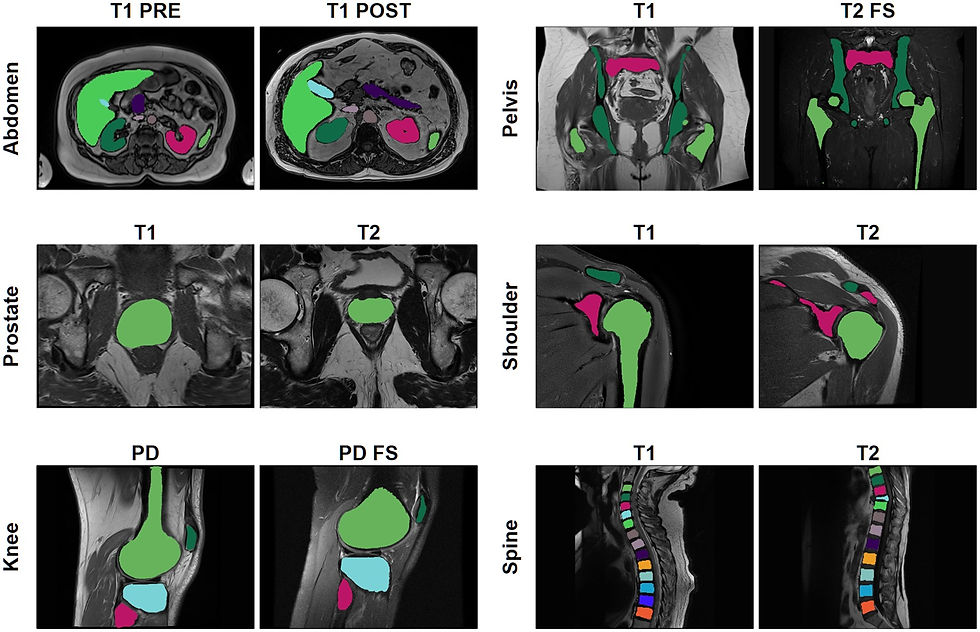
Automated MRI segmentation is essential for research and is increasingly integrated into clinical workflows for disease quantification and faster reporting. However, most existing models are trained for specific sequence types and anatomical regions, limiting their usability across diverse clinical applications. MRAnnotator is part of a growing trend toward generalizable deep learning models designed for multi-anatomy, multi-sequence segmentation. As reported in Radiology: Advances, the algorithm accurately identifies 44 anatomical structures across 8 regions in a wide range of MRI sequences.
MRAnnotator was trained on over 1,500 MRI sequences from 843 patients across multiple clinical sites. The algorithm demonstrated strong generalization, achieving Dice scores of 0.878 and 0.875 on internal and external test sets, respectively. It was benchmarked against two multi-anatomy segmentation models, TotalSegmentator MRI and MRSegmentator, as well as an nnU-Net trained specifically for abdominal segmentation. MRAnnotator consistently outperformed both multi-anatomy models while achieving comparable performance to the abdominal-specific nnU-Net.
Despite distribution shifts across scanner types and acquisition parameters, MRAnnotator maintained high segmentation accuracy, reinforcing its potential for radiology workflows, dataset curation, and AI model development. The ability to perform segmentation across multiple anatomies and sequences could enable the development of more quantitative tools that meet a broader range of clinical needs. The algorithm is publicly available, providing future researchers with a valuable tool to further refine multi-anatomy segmentation approaches.
MASAI trial: Detection Rates Up, Workloads Down
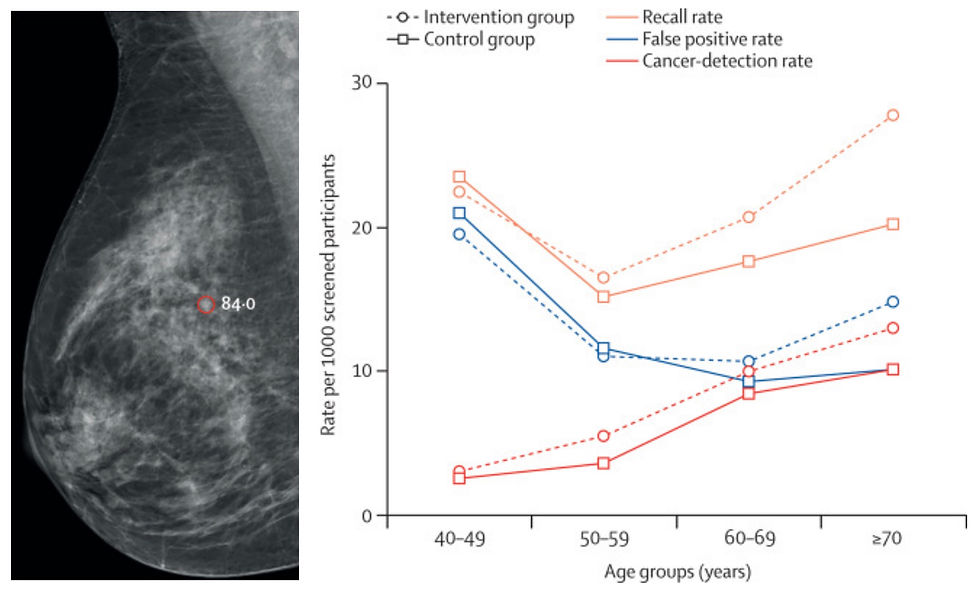
With medical imaging usage increasing and continued radiology workforce shortages, radiologist burnout rates are up. Many who once feared AI may take their job are now looking to AI to lighten their workload, especially for high volume routine procedures. This is particularly pertinent for breast cancer screening in Europe, where guidelines recommend double screen-reading. A recent Lancet Digit Health article provided the latest updates on the MASAI trial, a large-scale randomized controlled study evaluating the impact of AI-supported double reading within Sweden’s national mammography screening program.
The study compared traditional double reading to AI-supported double reads using ScreenPoint’s Transpara algorithm for over 100,000 women, finding that AI significantly increased breast cancer detection while reducing radiologists’ workload. AI support led to a 29% higher cancer detection rate (6.4 vs. 5.0 per 1000 screenings) without increasing false positives. Additionally, the AI-supported workflow reduced screen-reading workload by 44%, addressing workforce shortages in breast radiology without compromising accuracy. Notably, the AI system also identified more small, lymph-node negative, invasive cancers, suggesting potential for earlier clinical detection and intervention.
These findings are a huge win for ScreenPoint and highlight AI’s potential to both enhance screening efficiency and improve early cancer detection rates. The MASAI trial supports AI’s role in reducing missed diagnoses and streamlining mammography workflows, offering a scalable solution to staffing challenges. Future long-term follow-ups will further clarify AI’s impact on interval cancers and overall patient outcomes, but early results suggest that AI integration in breast cancer screening could be a transformative step toward a more efficient and effective radiology ecosystem.
New Dataset Alert: 100 Low-Field Neonatal Brain Scans
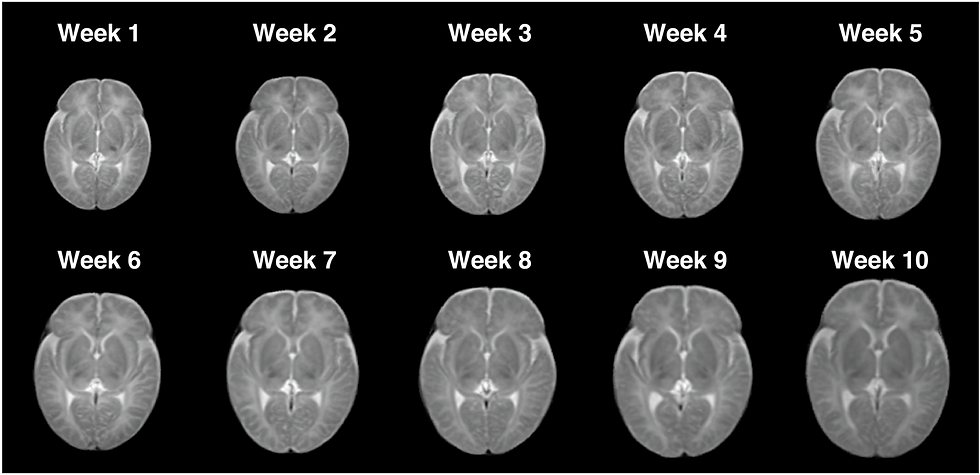
Low-field MRI is emerging as a cost-effective alternative for neonatal brain imaging, particularly where high-field MRI is impractical. A recent Scientific Data study introduces a dataset of 100 low-field MRI scans from infants aged 1 to 70 days. Scans were acquired on the recently FDA cleared 0.35T Nido scanner from LiCi Medical. All sequences are T2 with 0.85 mm in-plane resolution and 6 mm slice thickness. The study also demonstrates the utility of the data by performing segmentation, creating a spatiotemporal atlas, and replicating known developmental asymmetries and sex-based differences. The data is available for access after completing a data use agreement.
New Dataset Alert: fastMRI Breast
The fastMRI datasets have become a cornerstone of accelerated MRI research, and their offerings continue to expand. In Radiology: Artificial Intelligence, researchers announced the release of the fastMRI Breast dataset, the largest public collection of k-space and DICOM data for breast dynamic contrast-enhanced MRI. Like previous fastMRI datasets, it aims to support advancements in machine learning-based image reconstruction but can also benefit a range of research projects.
The dataset includes 300 imaging studies from 284 patients, along with case-level labels covering key clinical details such as lesion status, tumor grade, and biomarkers. Unlike most existing MRI datasets that provide only reconstructed images, this dataset includes raw k-space data, allowing researchers to develop novel reconstruction techniques and quantitative imaging approaches. To enhance accessibility and reproducibility, it also includes open-source reconstruction code.
Resource Highlight
Interested in pursuing a PhD in low-field MRI? King’s College London, a leading institution in low-field MRI research, is accepting applications to study low-field MRI in dementia patients. You'll be mentored by Ashwin Venkataraman, František Váša, and Steve Williams—the authors behind the feature article in the very first edition of RadAccess! This is an excellent opportunity to explore low-field MRI in depth, but you have to apply NOW—applications close February 14th!
Feedback
We’re eager to hear your thoughts as we continue to refine and improve RadAccess. Is there an article you expected to see but didn’t? Have suggestions for making the newsletter even better? Let us know! Reach out via email, LinkedIn, or X—we’d love to hear from you.
References
Zhang, Sheng, et al. "A Multimodal Biomedical Foundation Model Trained from Fifteen Million Image–Text Pairs." NEJM AI 2.1 (2025): AIoa2400640.
Zhou, Alexander, et al. "MRAnnotator: multi-anatomy and many-sequence MRI segmentation of 44 structures." Radiology Advances 2.1 (2025): umae035.
Hernström, Veronica, et al. "Screening performance and characteristics of breast cancer detected in the Mammography Screening with Artificial Intelligence trial (MASAI): a randomised, controlled, parallel-group, non-inferiority, single-blinded, screening accuracy study." The Lancet Digital Health (2025).
Sun, Zhexian, et al. "A Low-Field MRI Dataset For Spatiotemporal Analysis of Developing Brain." Scientific Data 12.1 (2025): 109.
Solomon, Eddy, et al. "fastMRI Breast: A publicly available radial k-space dataset of breast dynamic contrast-enhanced MRI." Radiology: Artificial Intelligence 7.1 (2025): e240345.
Disclaimer: There are no paid sponsors of this content. The opinions expressed are solely those of the newsletter authors, and do not necessarily reflect those of referenced works or companies.