- Campbell Arnold
- Mar 18
- 3 min read
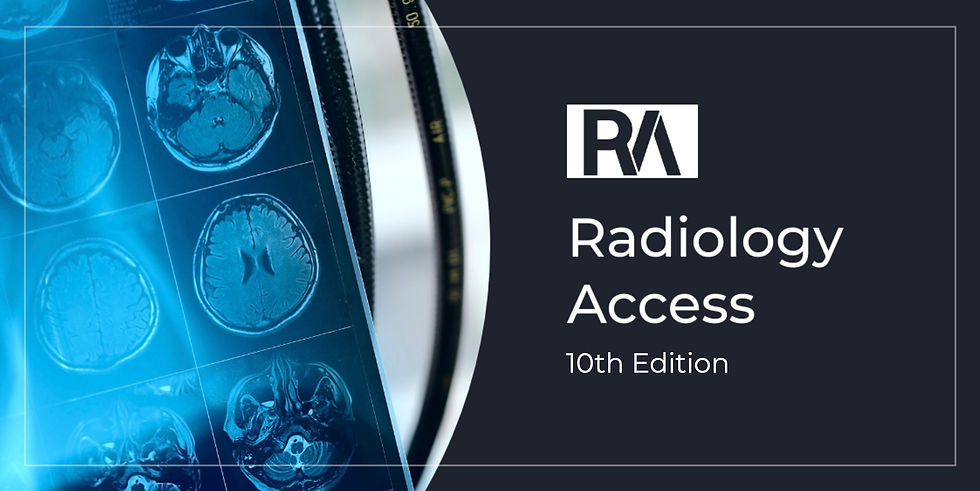
Welcome to RadAccess: Impressions—your quick-read companion to the main RadAccess newsletter. Like a radiology report's Impressions section, here we deliver the essential information concisely, respecting your time. For more details, you can always turn to the full RadAccess newsletter.
Zero-to-Hero: AI-Guided Ultrasound Enables Non-Expert Users
Portable ultrasound is expanding access to medical imaging in new areas and context, however expert sonographers aren't readily available in many of these situation. Could AI help bridge the training gap? A recent study in NPJ Digital Medicine evaluated ThinkSono’s AI-guided ultrasound system, which helps non-experts perform compression ultrasound for deep vein thrombosis (DVT) diagnosis. The study included 381 patients from across 11 UK hospitals and compared expert sonographer exams to AI-assisted scans by non-ultrasound-trained nurses using a portable Clarius ultrasound. 80% of AI-guided scans were deemed diagnostic quality and clinical review showed high sensitivity (90-98%) and negative predictive value (98-99%), indicating AI-guidance can enable reliable DVT assessments by non-expert imagers. The system also reduced the need for expert-led scans by 29-53%, which offers a potential solution to sonographer shortages and expanding ultrasound access, particularly in underserved areas.
AI Takes the Call: Automating Emergency MRI Protocoling
With rising imaging volumes, radiologists must spend more time on protocol selection—a critical but also mundane task which interrupts their workflow and decreases efficiency. A recent Radiology: Artificial Intelligence article explored the use of AI models for automating MRI protocol selection. They study analyzed 1,953 emergency brain MRI referrals collected over 3 years and evaluated protocol suggestions from five AI models—Naïve Bayes, Support Vector Machine, XGBoost, BERT, and GPT-3.5. The algorithms used referral text to classify requests into 12 protocols and determine whether contrast should be used. GPT-3.5 performed best, achieving 84% accuracy for protocol selection and 91% for contrast determination, matching the performance of emergency radiologists. The findings suggest that NLP-based AI models could help streamline protocoling, reducing radiologists' cognitive load while maintaining accuracy.
MICCAI LISA Challenge Results: Advancing AI for Low-field MRI
Ultra-low-field MRI offers a cost-effective and portable alternative to traditional MRI, but its lower image quality and the shortage of trained personnel present significant barriers to widespread adoption. The LISA Challenge held at MICCAI aimed at addressing these issues using AI-driven solutions. The competition focused on two key tasks: automated image quality assessment and hippocampal segmentation. The challenge attracted 36 teams from nine countries, with participants working with two datasets collected across sites in South Africa, Pakistan, and Uganda, including 648 low-field MRI scans with seven artifact types for quality assessment and 99 paired high-field/low-field scans with manual hippocampal segmentations.
The results for the competition were recently published in Lecture Notes in Computer Science. In the quality assessment task, the top-performing model achieved 82.3% accuracy using a DenseNet-based architecture trained with simulated artifacts for enhanced robustness. For the hippocampal segmentation task, the leading algorithm was a 3D nnUNet which acheived a Dice score of 0.72. Additional details on the top three algoirhtm for each task, along with github links, is available here. The success of these AI models highlights the potential for automated quality control and anatomical segmentation to make low-field MRI more practical, reducing training requirements and expanding imaging access in underserved regions.
Deep Reconstruction Workshop at JHU (Free In-Person & Virtual)
The FREE Deep Reconstruction Workshop will be held March 22-23 at Johns Hopkins University, hosted by J. Webster Stayman. This event brings together top experts to discuss deep learning-based image reconstruction across MRI, CT, PET, and ultrasound. Open to both in-person and virtual attendees—register today to secure your spot!
NVIDIA GTC 2025 in San Jose (Free Virtual)
Free NVIDIA conference happening NOW! This year NVIDIA’s GTC AI Conference runs from March 17-21. The conference features keynotes, technical sessions, and cutting-edge AI research across healthcare, robotics, and more. Virtual attendance is free—register now!
Feedback
We’re eager to hear your thoughts as we continue to refine and improve RadAccess. Is there an article you expected to see but didn’t? Have suggestions for making the newsletter even better? Let us know! Reach out via email, LinkedIn, or X—we’d love to hear from you.
References
Speranza, Giancarlo, et al. "Value of clinical review for AI-guided deep vein thrombosis diagnosis with ultrasound imaging by non-expert operators." npj Digital Medicine 8.1 (2025): 135.
Huhtanen, Heidi J., et al. "Machine Learning and Deep Learning Models for Automated Protocoling of Emergency Brain MRI Using Text from Clinical Referrals." Radiology: Artificial Intelligence (2025): e230620.
Lepore, Natasha, and Marius George Linguraru. "Low field pediatric brain magnetic resonance image segmentation and quality assurance." 27th International Conference on Medical Image Computing and Computer Assisted Intervention (MICCAI 2024). Zenodo. 2024.
Disclaimer: There are no paid sponsors of this content. The opinions expressed are solely those of the newsletter authors, and do not necessarily reflect those of referenced works or companies.