- Campbell Arnold
- Apr 1
- 4 min read
Updated: 5 days ago
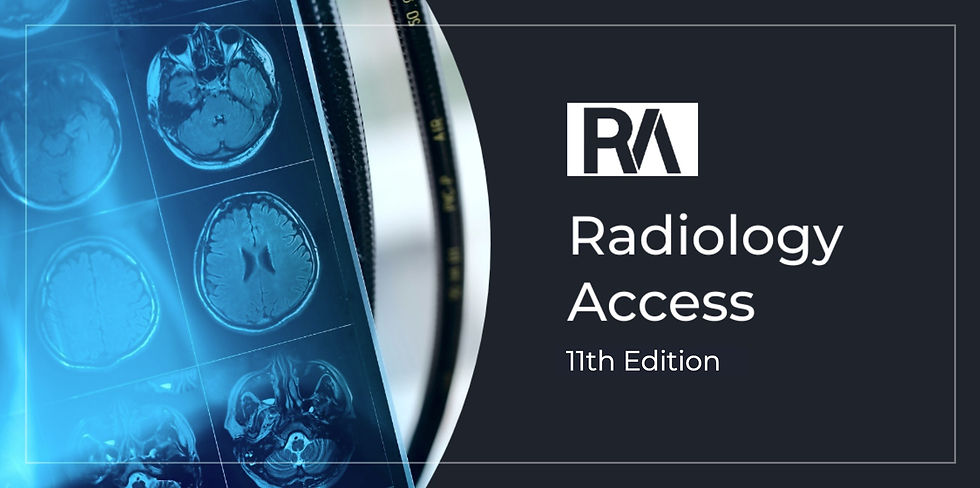
Welcome to RadAccess: Impressions—your quick-read companion to the main RadAccess newsletter. Like a radiology report's Impressions section, here we deliver the essential information concisely, respecting your time. For more details, you can always turn to the full RadAccess newsletter.
MedHELM: Comprehensive Benchmarking for Medical LLMs
While large language models (LLMs) like ChatGPT have excelled on medical exams, their real-world clinical reliability remains uncertain. To address this gap, researchers at Stanford and Microsoft developed MedHELM, a benchmarking framework that evaluates LLMs on clinically relevant tasks using real electronic health records. MedHELM assesses AI across five key categories—Clinical Decision Support, Evidence-Based Medicine, Biomedical Knowledge, Clinical Conversations, and Medical Reasoning—spanning 22 subcategories and 121 tasks. It integrates diverse datasets and multiple evaluation metrics, including accuracy, bias detection, and reliability in high-stakes environments. Notably, initial results showed that smaller models sometimes outperform state-of-the-art models in tasks like patient communication. MedHELM provides a more nuanced assessment of LLMs, guiding their responsible deployment in healthcare.
UniMed-CLIP: Improving Foundation VLMs for Medical Imaging
Vision-Language Models (VLMs) have shown great success in natural image tasks, but their adoption in healthcare thus far has been limited, with some models showing poor generalization to medical tasks (despite conveying high confidence!). To address this, researchers introduced UniMed-CLIP, a new VLM trained on a large-scale, open-source medical dataset containing 5.3 million image-text pairs across six imaging modalities (X-ray, CT, MRI, ultrasound, retinal fundus, and histopathology). The dataset was created using Large Language Models (LLMs) to convert image-label data into paired text descriptions. UniMed-CLIP outperforms generalist VLMs and rivals modality-specific models, achieving a 12.61% improvement over BiomedCLIP despite using significantly less training data. This highlights the importance of well-curated datasets in medical AI. The dataset, code, and model weights are open-source, with a demo available on Hugging Face.
Match Day 2025: Can Residency Growth Outpace the Radiologist Shortage?
Match Day 2025 saw radiology residency programs expand to a record 1,451 positions—a 5.2% increase from last year. However, applicants to diagnostic radiology programs declined for the second year in a row, dropping 6.4% to 1,759. The decline may be due in part to the specialty's perceived competitiveness and the potential for AI’s impact on future job prospects. Despite this, radiology maintained a strong 98.2% fill rate, with just 26 unfilled positions (likely to be filled through SOAP). The overall match rate was 81%, rising to 93% for US MD applicants. Interventional radiology saw record growth, with its largest-ever class of 207 residents and a 3% increase in applicants, reflecting sustained interest in procedural radiology. Unfortunately, while increasing residency spots is a positive step, it will at best keep pace with imaging demand rather than significantly addressing the current radiologist shortage.
Promaxo’s Low-Field MRI System Showcases Diagnostic Potential
Prostate cancer detection relies on accurate biopsies, with MRI offering superior lesion visualization over ultrasound. However, high-field MRI is expensive and impractical for in-office procedures. Promaxo's single-sided low-field MRI addresses this gap by combining real-time registration with high-field images, making MRI-guided biopsies more accessible to urologists. With over 150 units sold, Promaxo reports a 77% cancer detection rate and a 23% increase in detecting clinically significant cancers. A recent Bioengineering study found that quantitative texture analysis on low-field MRI could distinguish cancerous from normal tissue, reinforcing its potential as a cost-effective, accessible tool for prostate cancer detection and biopsy planning.
New Radiology AI Research Journal with Limited Time Free Publication
The European Journal of Radiology has launched a new open-access sister journal, European Journal of Radiology Artificial Intelligence (EJR AI), dedicated to AI applications in clinical radiology. Led by Pascal Baltzer (Medical University of Vienna) and Matthias Dietzel (University Hospitals Erlangen), EJR AI aims to advance research in radiology AI. Notably, there is no Article Publishing Charge for submissions made before September 29, 2026, making it free to publish for the next 18 months—a great opportunity for researchers looking to share their work without publication fees.
Feedback
We’re eager to hear your thoughts as we continue to refine and improve RadAccess. Is there an article you expected to see but didn’t? Have suggestions for making the newsletter even better? Let us know! Reach out via email, LinkedIn, or X—we’d love to hear from you.
References
Kung, Tiffany H., et al. "Performance of ChatGPT on USMLE: potential for AI-assisted medical education using large language models." PLoS digital health 2.2 (2023): e0000198.
Bhayana, Rajesh, Satheesh Krishna, and Robert R. Bleakney. "Performance of ChatGPT on a radiology board-style examination: insights into current strengths and limitations." Radiology 307.5 (2023): e230582.
https://hai.stanford.edu/news/holistic-evaluation-of-large-language-models-for-medical-applications
Khattak, Muhammad Uzair, et al. "Unimed-clip: Towards a unified image-text pretraining paradigm for diverse medical imaging modalities." arXiv preprint arXiv:2412.10372 (2024).
Le, Dang Bich Thuy, et al. "Haralick Texture Analysis for Differentiating Suspicious Prostate Lesions from Normal Tissue in Low-Field MRI." Bioengineering 12.1 (2025): 47.
https://www.sciencedirect.com/journal/european-journal-of-radiology-artificial-intelligence
Disclaimer: There are no paid sponsors of this content. The opinions expressed are solely those of the newsletter authors, and do not necessarily reflect those of referenced works or companies.