- Campbell Arnold
- Feb 18
- 3 min read
Updated: Mar 27
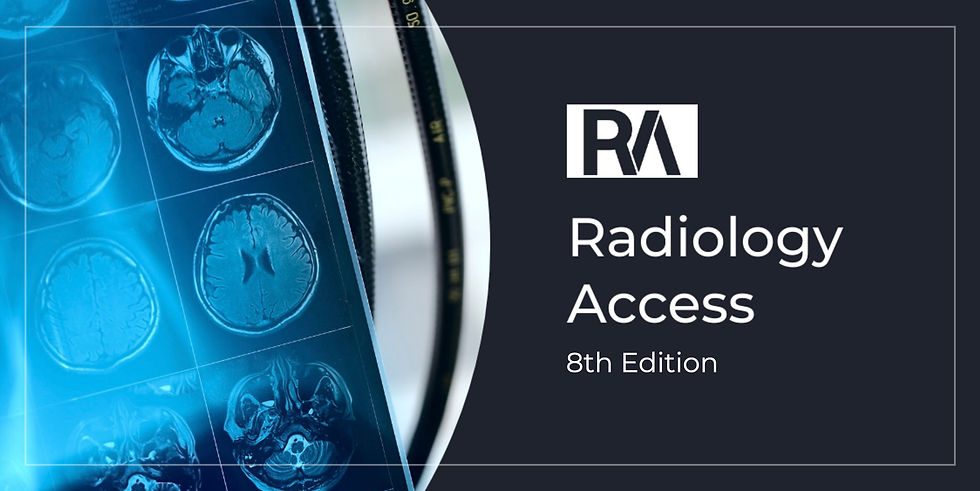
Welcome to RadAccess: Impressions—your quick-read companion to the main RadAccess newsletter. Like a radiology report's Impressions section, here we deliver the essential information concisely, respecting your time. For more details, you can always turn to the full RadAccess newsletter.
Microsoft’s Open-Access Foundation Imaging Model: BiomedCLIP
Microsoft continues to expand its presence in biomedical AI with BiomedCLIP, a vision-language model introduced in NEJM AI. BiomedCLIP builds on OpenAI’s CLIP framework by training a biomedical specific model using over 15 million image-text pairs from 3 million PubMed articles. BiomedCLIP spans 30+ imaging categories and outperforms existing models in image retrieval, classification, and medical question-answering. It even surpassed a radiology-specific model on RSNA’s pneumonia detection challenge. BiomedCLIP can reduce the need for extensive labeled datasets with zero-shot and few-shot learning, further accelerating AI development in radiology.
MRAnnotator: Seeing the Big Picture
Automated MRI segmentation is crucial for research and clinical workflows, yet most models are limited to specific sequences or anatomies. MRAnnotator, introduced in Radiology: Advances, takes a step towards generalized segmentation–spanning 44 structures across 8 anatomical regions and multiple sequences. The algorithm was trained on 1,500 scans from 843 patients and outperformed existing multi-anatomy models while matching the performance of an anatomy specific algorithm. Despite variations in scanners and acquisition parameters, MRAnnotator maintained high accuracy, highlighting its potential for integration into real-world clinical workflows and development of more robust segmentation-based quantitative tools.
MASAI trial: Detection Rates Up, Workloads Down
With rising imaging volumes and radiologist shortages, AI is increasingly seen as a tool to ease workloads. This is particularly salient in high-volume screening programs like double-read breast cancer screening in Europe. A Lancet Digit Health article provides the latest update on the MASAI trial, which is evaluating AI-supported double reading in Sweden’s national mammography program. Over 100,000 screenings were randomized to traditional double-reads or AI-supported double-reads with ScreenPoint’s Transpara algorithm. The AI algoirthm increased cancer detection by 29% and reduced radiologists’ workload by 44%, all without raising false positives. There are even preliminary signs the algoirthm may improve early cancer detection. Truly stellar results! This is huge win for ScreenPoint and will likely drive further adoption in Europe.
New Dataset Alert: 100 Low-Field Neonatal Brain Scans
While interest in low-field MRI research has rapidly grown, there is still a paucity of available datasets. A recent Scientific Data study presents a dataset of 100 low-field MRI scans from infants (1–70 days old) acquired on the recently FDA cleared 0.35T Nido scanner from LiCi Medical. The data is available with a data use agreement.
New Dataset Alert: fastMRI Breast
The fastMRI datasets have been instrumental in MR acceleration research. Their latest addition, the fastMRI Breast dataset, further expands this impact. Published in Radiology: Artificial Intelligence, it is the largest public collection of k-space and DICOM data for breast dynamic contrast-enhanced MRI, featuring 300 studies from 284 patients with case-level clinical labels. They also include open-source reconstruction code alongside the dataset.
Feedback
We’re eager to hear your thoughts as we continue to refine and improve RadAccess. Is there an article you expected to see but didn’t? Have suggestions for making the newsletter even better? Let us know! Reach out via email, LinkedIn, or X—we’d love to hear from you.
References
Zhang, Sheng, et al. "A Multimodal Biomedical Foundation Model Trained from Fifteen Million Image–Text Pairs." NEJM AI 2.1 (2025): AIoa2400640.
Zhou, Alexander, et al. "MRAnnotator: multi-anatomy and many-sequence MRI segmentation of 44 structures." Radiology Advances 2.1 (2025): umae035.
Hernström, Veronica, et al. "Screening performance and characteristics of breast cancer detected in the Mammography Screening with Artificial Intelligence trial (MASAI): a randomised, controlled, parallel-group, non-inferiority, single-blinded, screening accuracy study." The Lancet Digital Health (2025).
Sun, Zhexian, et al. "A Low-Field MRI Dataset For Spatiotemporal Analysis of Developing Brain." Scientific Data 12.1 (2025): 109.
Solomon, Eddy, et al. "fastMRI Breast: A publicly available radial k-space dataset of breast dynamic contrast-enhanced MRI." Radiology: Artificial Intelligence 7.1 (2025): e240345.
Disclaimer: There are no paid sponsors of this content. The opinions expressed are solely those of the newsletter authors, and do not necessarily reflect those of referenced works or companies.